reasoning压缩(一)
【本文已在同名 微信公众号 / 知乎 / 个人博客linsight.cn 上线】
之前讲过了prompt压缩,追求把模型的输入减少一些:
而如今长思考模型的使用越来越多,模型的输出长度也成了一个问题,于是也就有了压缩思考过程的尝试。
Token-Budget-Aware LLM Reasoning
寻找最佳budget
这篇文章里,他们发现了在prompt里加上生成budget的限制,比如要求模型使用50个token以内来回答问题,会改变模型生成的长度。比如下面这个例子:
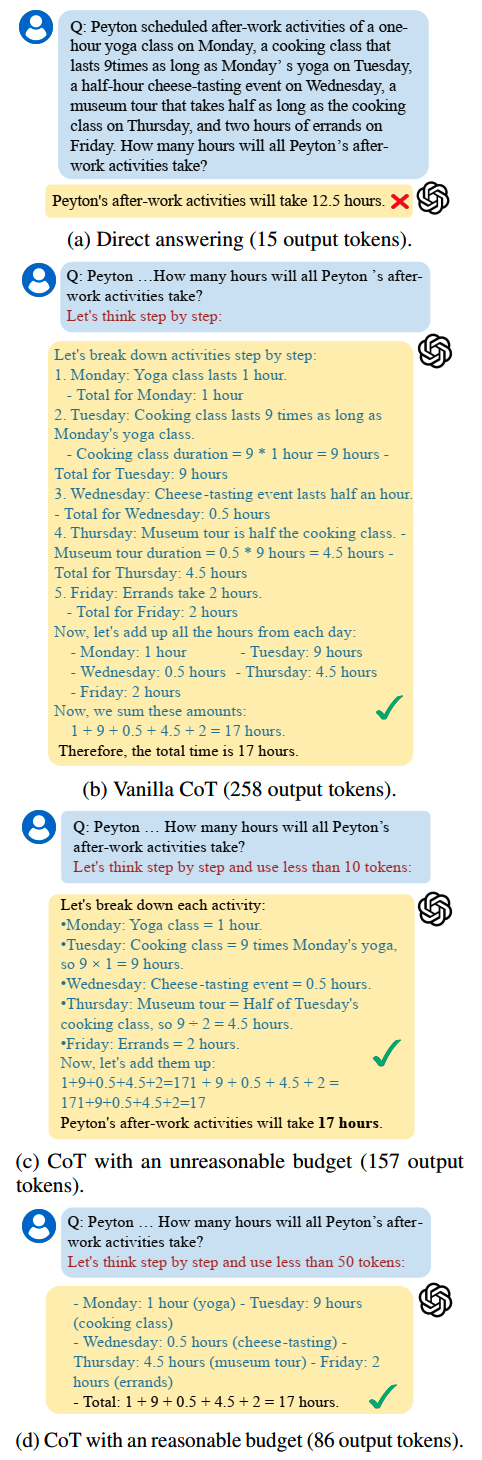
但是这个budget的选择也有讲究。首先,不合适的budget会影响模型的效果,准确率会受影响。另外,如果选择一个过低的budget,模型的生成长度上反而比合理budget下要长。比如上面这个例子,当budget=50的时候,模型最终生成长度为86;而如果把budget降到10,模型生成的长度就反而增长到157。文中把这个现象叫做token elasticity phenomenon。当给定的budget低于合理的范围,就会出现这个不减反增的现象。
因此怎么选择一个好的budget,在保证效果的情况下,又能让模型减少输出长度,就是关键所在。
文中首先用二分法来搜索一个budget,来保证模型输出的正确性。具体来说,就是用原CoT作为budget搜索的右边界(right),0作为初始的左边界(left)。如果在budget=(left + right)/2的情况下模型输出依然正确,说明budget还能再压缩,那下一步就搜索左边,反之就要增加budget,去搜索右边:
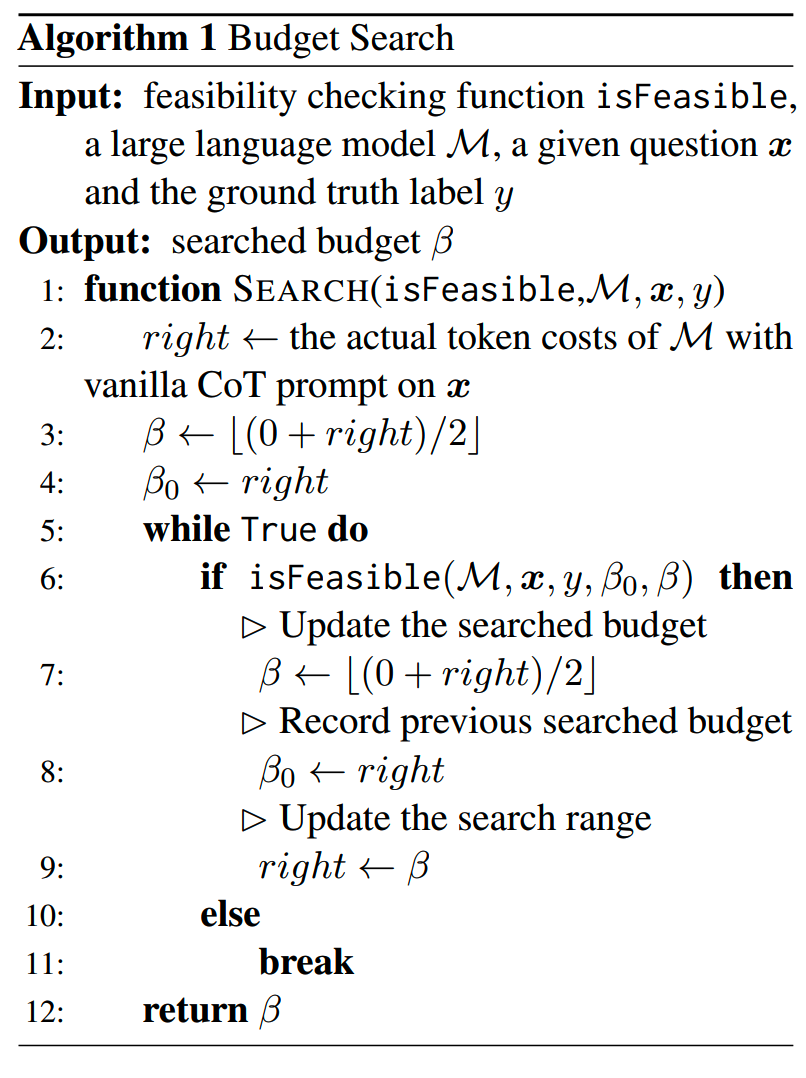
为了对付token elasticity phenomenon的问题,算法1里的isFeasible里用贪心策略,要求当前budget下的实际输出长度必须要比上次的短:
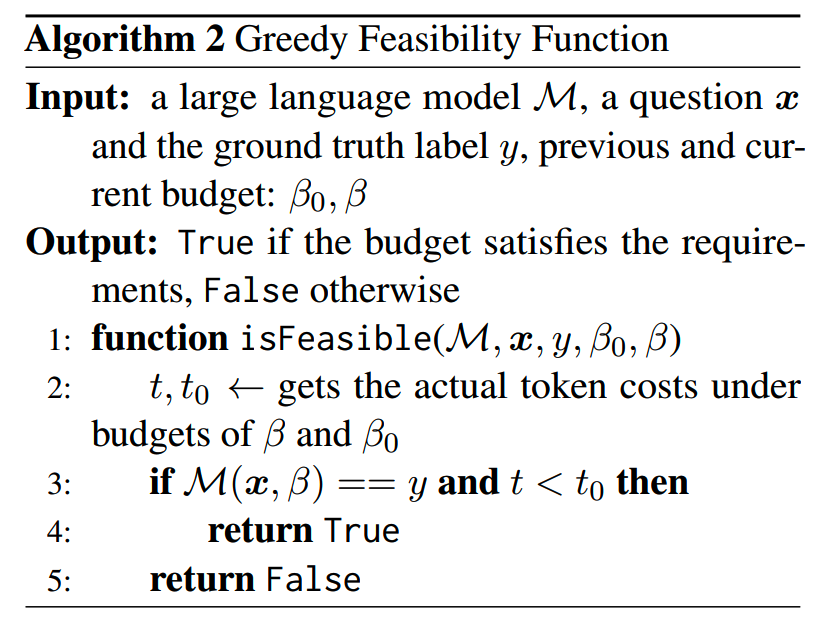
TALE-EP
基于前面的分析,文中提出两个方法来压缩reasoning长度,第一个是TALE-EP。
TALE = Token-budget-Aware Llm rEasoning
EP = Estimation and Prompting
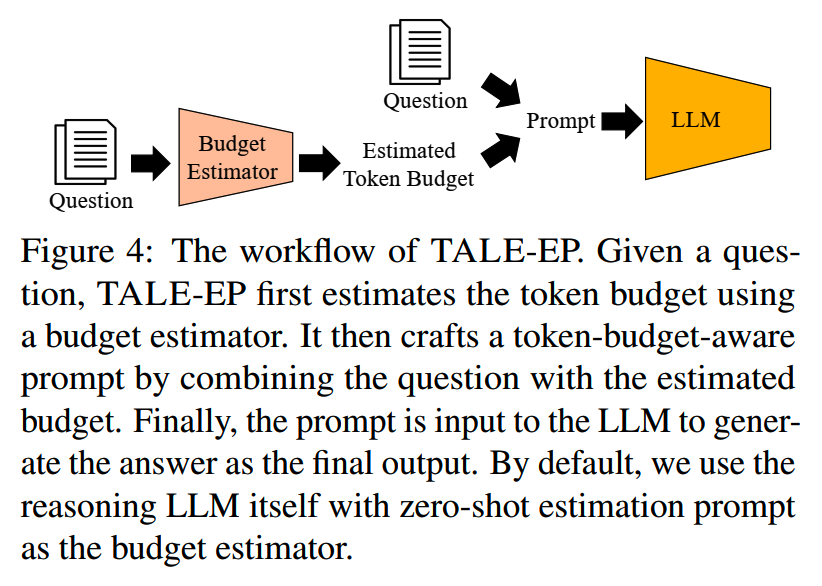
预测budget部分则是使用一个LLM,用下面的prompt来给出预测:
1 | Task: Analyze the given question and estimate the |
再看一个TALE-EP的例子:
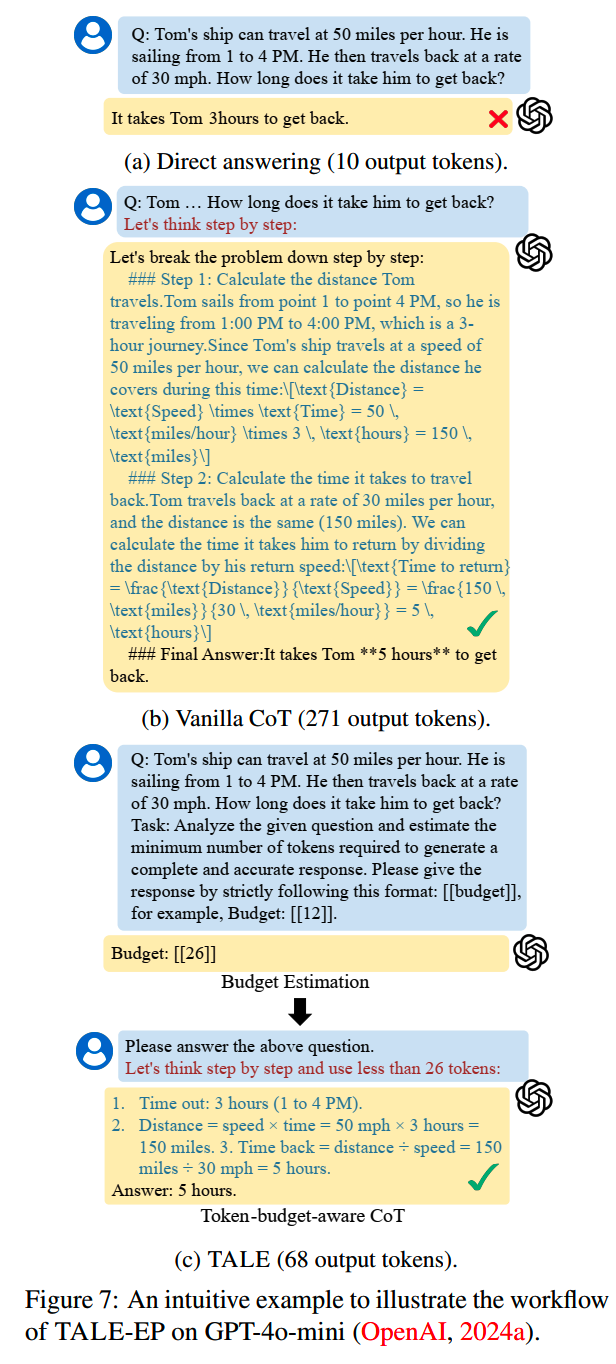
TALE-EP不需要训练模型,只需要依赖LLM+prompt对budge进行预测。
TALE-EP能比CoT减少67%左右的token(从Vanilla CoT的461.25 → 148.72 tokens),不过效果也比CoT稍微差一点点(83.75% → 81.03%)。
TALE Post-Training (TALE-PT)
另一个方式TALE-PT,则是直接把token的压缩训练到生成模型中去,相当于让生成模型自己隐式地去判断应该要用多少budget。训练方式有SFT和DPO两种。
1、SFT
把原来的CoT数据按前面的algorithm1和2改造成短思考的形式,然后直接微调生成模型。
2、DPO
另外一个方法就是使用强化学习DPO,用改造过的短思考数据作为正例,而原CoT的答案作为负例,进行训练。
经过SFT训练的TALE-PT效果还是比没有训练的TALE-EP更好一些:
- SFT版:+1.01%(77.56% → 78.57%)
- DPO版:-3.45%(77.56% → 74.11%),token也减少了50%左右。
Chain of Draft(CoD)
CoT要求模型一步一步分析当前的问题,把复杂问题分解成可以快速解决的子问题。这种要求下,模型的输出其实就是在碎碎念:“我现在要煮一个水煮牛肉,先切牛肉……逆纹切薄片才嫩,这块肉怎么这么难切?刀该磨了……料酒、生抽、淀粉、蛋清……蛋清是不是放多了?算了先这样试试吧……腌二十分钟够不够?现在再尝试加点料酒……”
这样虽然能够让过程清晰一些,但是也显得有些啰嗦了。
CoD就参照人类专业一些的做法。比如学霸在做数学题的时候可能就不会碎碎念,而是首先把当前的条件都用简洁的方式表达出来,然后列几个候选方案的公式,最后验证结果。
因此相比CoT,CoD在system prompt上就明确要求用更少的文字来进行思考:
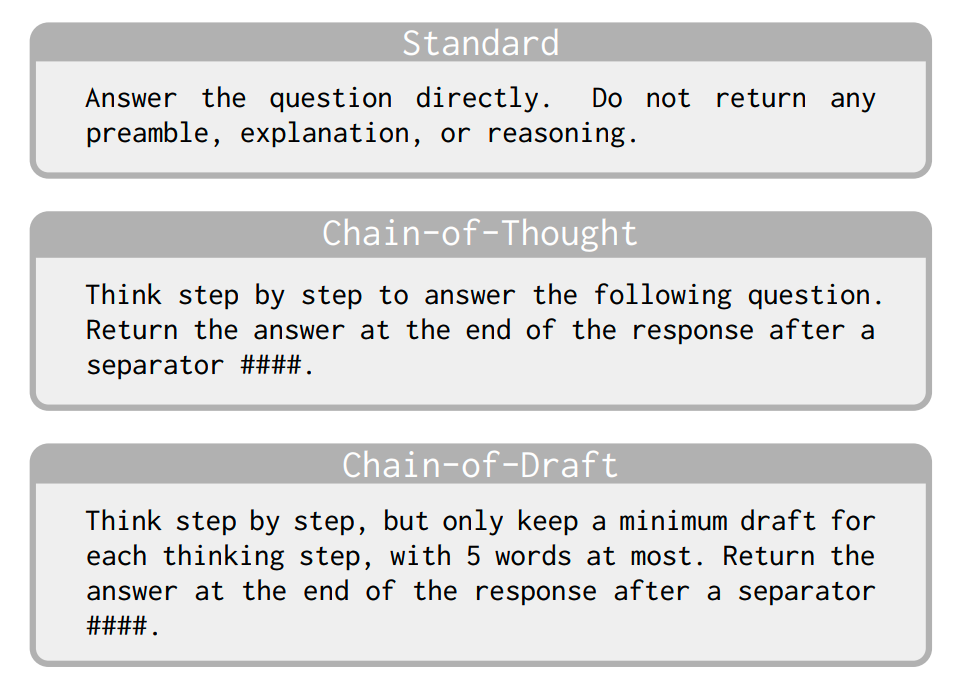
另外CoD还会提供一些人工编写,包含简洁思考过程的case来作为示例。比如用于GSM8K的system prompt和few-shot example是这样的:
1 | system_prompt: | |
CoD基本上需要给每个任务提供不同的few-shot example,这里还是有些工作量的。其他任务的prompt都能在论文github找到。
这些example很重要,原文发现没有使用这样示例样本,CoD的准确率就会大打折扣,甚至比不用CoT直接回答高不了多少:
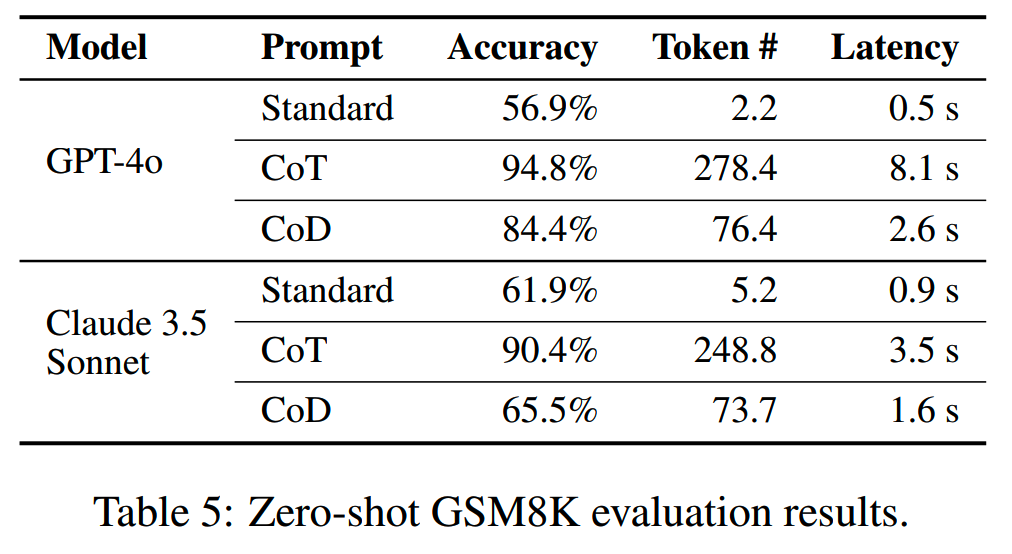
在使用较大模型时,在压缩reasoning长度上,CoD的效果还是不错的,最大能压缩到CoT的7%,而准确率还能基本持平:
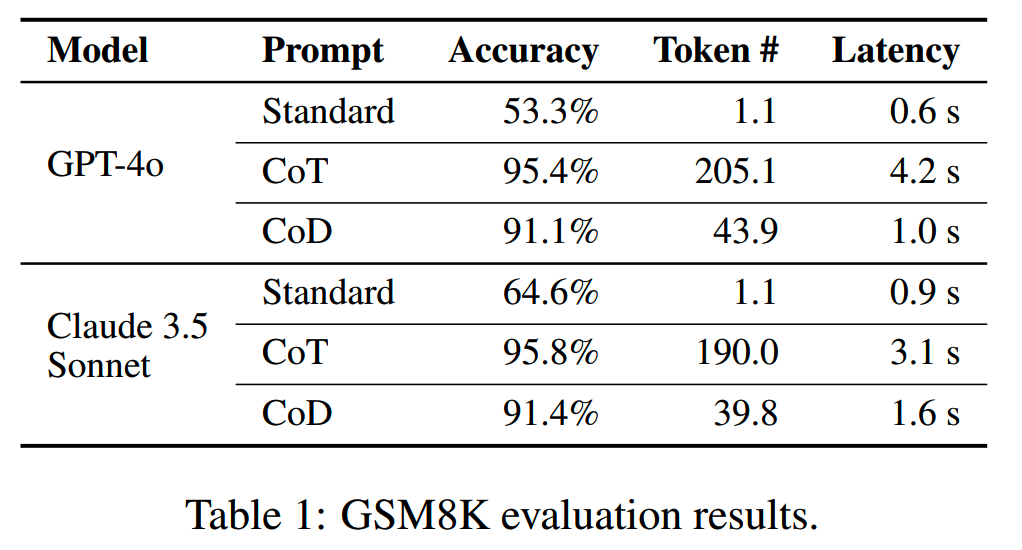
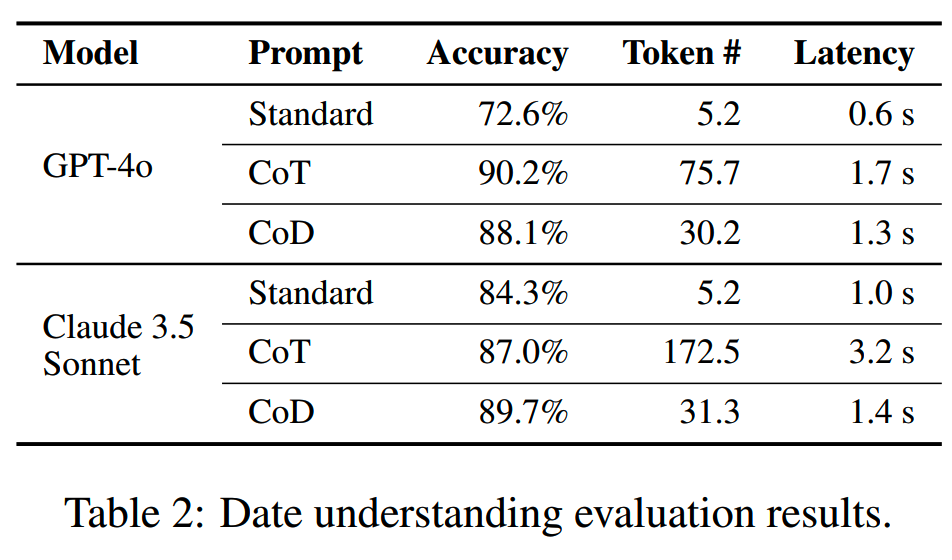
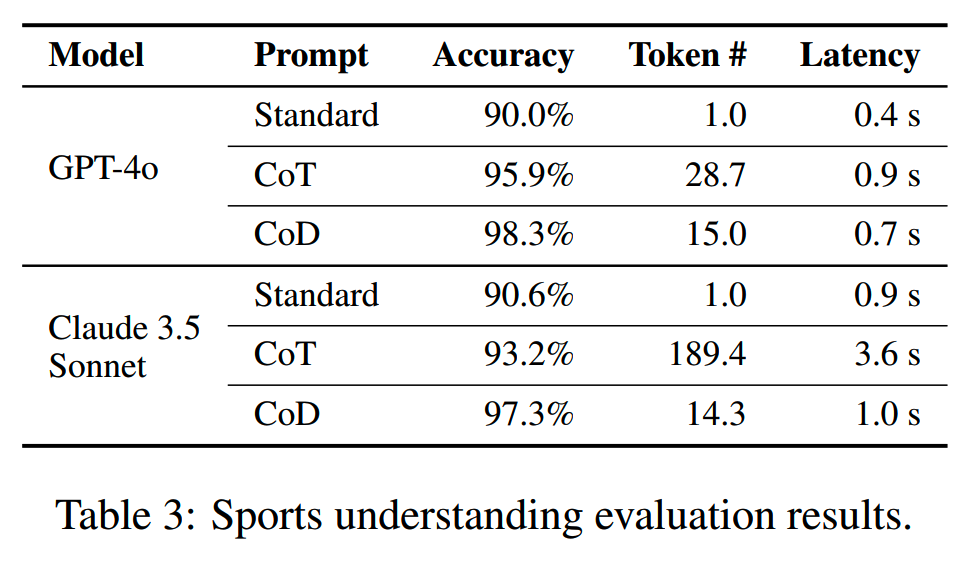
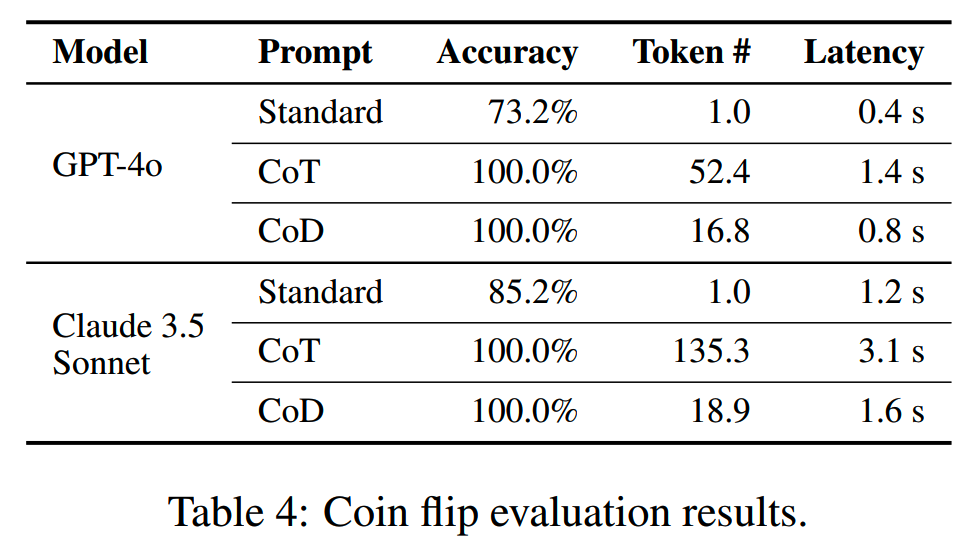
不过在小模型上(1.5B、3B之类的)效果就不行了:
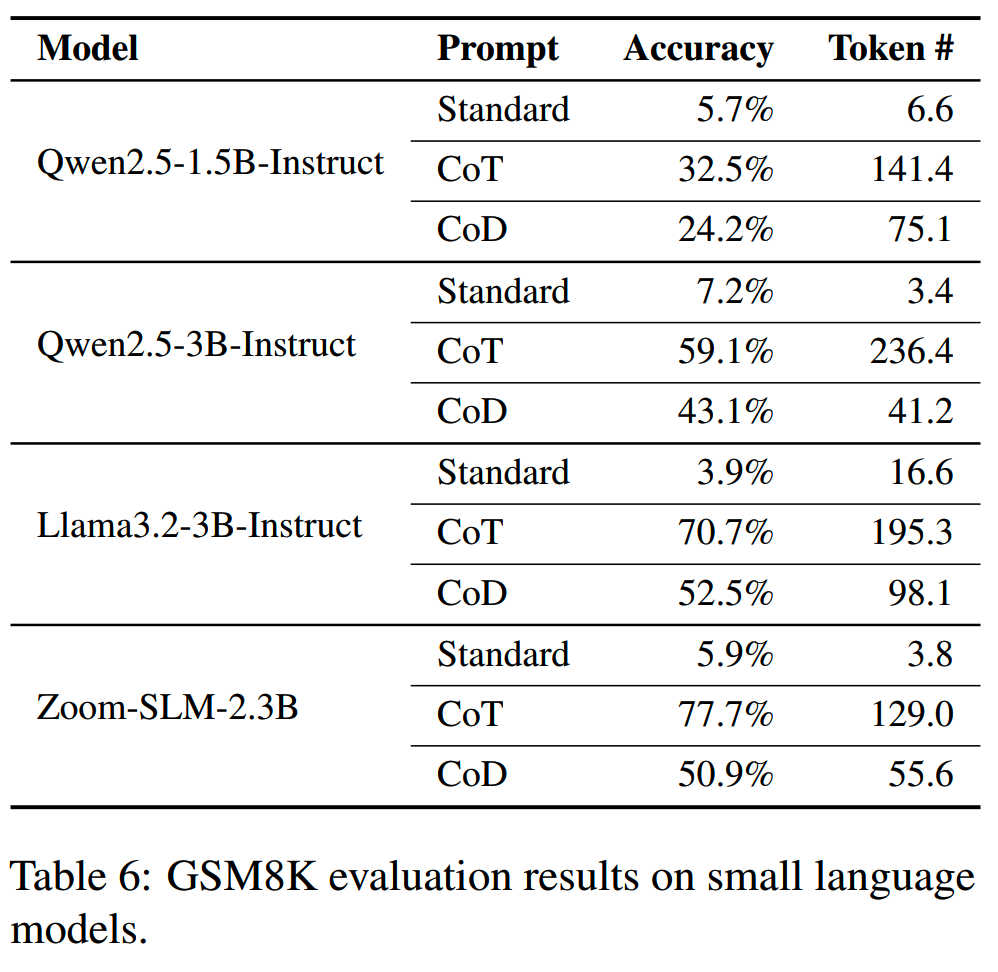
Sketch-of-Thought(SoT)
专业思维
SoT和CoD的大思路其实有些相似(名字也相关,sketch和draft嘛),都是认为CoT的碎碎念没有必要,应该用更加专业的人类思维方式来进行思考,不用啥都往思维链里放。
在这个framework下,SoT自己先提出三种抽象的专业思维方式:
- Conceptual Chaining
- Chunked Symbolism
- Expert Lexicons
1、Conceptual Chaining
Conceptual Chaining extracts essential terms and presents reasoning as direct step-by-step pathways with minimal text
Conceptual chaining仿照人类对事物概念的快速联想,用最简洁的符号来表示这种联想,比如箭头→。这种形式不用太关注这个联想的具体关系是什么,比如看到雨你可以联想到伞,那就“雨→伞”,也可以联想到云,那就“雨→云”,也可以联想到树,“雨→树”。这种方式不用把二者的具体关系都碎碎念解释出来,但是也很符合人类的思考方式。
Conceptual chaining适合用于commonsense reasoning相关的任务:
1 | Q: What is the name of the currency used in Seoul? |
具体的prompt是这样的:
1 | ## **Role & Objective** |
这个prompt主要分为三部分:
- 第一部分:角色定位和目的说明
- 第二部分:介绍Conceptual Chaining和它适用的场景
- 第三部分:具体说明怎么使用Conceptual Chaining来解决问题,都有什么细节要关注
其他的prompt在https://github.com/ashishpatel26/sot/tree/main/sketch_of_thought/config/prompts可以找到。
2、Chunked Symbolism
人类看信息的时候会把信息分块,比如一段段看,或者读电话号码的时候,会把3个或者4个数字看做一个chunk。
放到reasoning里,就是让模型将复杂的数学推理过程拆解为更小的、可管理的chunk,并通过符号和公式紧凑表达,避免自然语言的冗余描述。
1 | Q: A car accelerates at 2.5 m/sˆ2 for 10 seconds. If |
3、Expert Lexicons
缩写这个大家都很熟悉,在特定领域都有大量的专有缩写,这让我们可以减少很多冗余文本的使用。
1 | Q: A patient with STEMI is given MONA therapy. They |
Router
以上这几种思考方式分别适合用于不同的场景。
Conceptual Chaining适用于常识推理、多跳推理、Fact-based Recall。而Chunked Symbolism就适用于数学推理和符号逻辑问题。Expert Lexicons则适合专业领域比如医学相关的推理。
因此需要一个router根据输入问题的不同,选择一个适合的prompt来处理。文中训练了一个DistilBERT来做这个分流,DistilBERT模型比较小效率很高。训练数据来自于14200条reasoning task的数据,这些数据用GPT-4o + prompt打标,分成这三类中的一类。
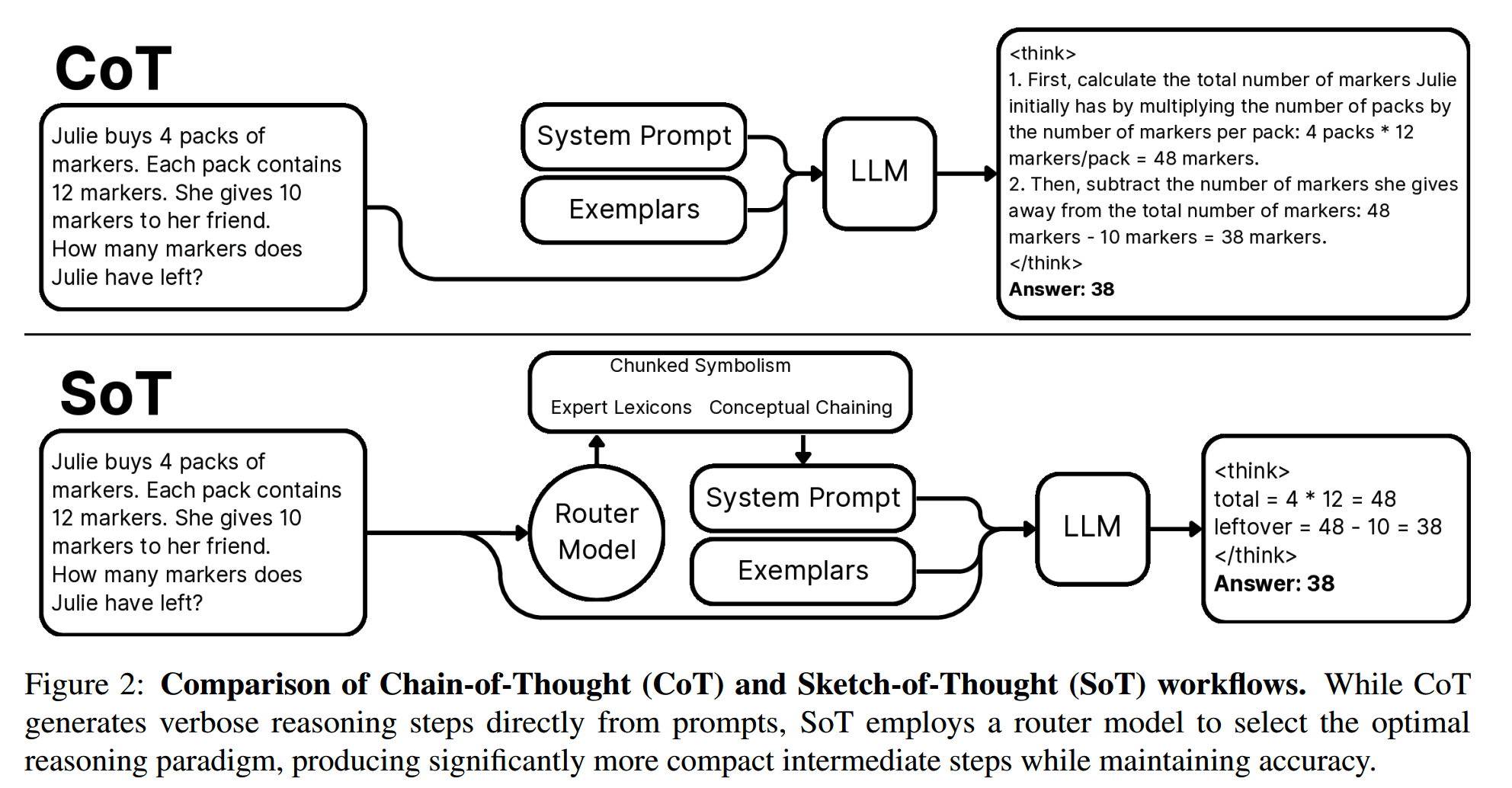
效果
相比CoT,SoT在效果差不多的情况下,可以减少70%左右的token使用。
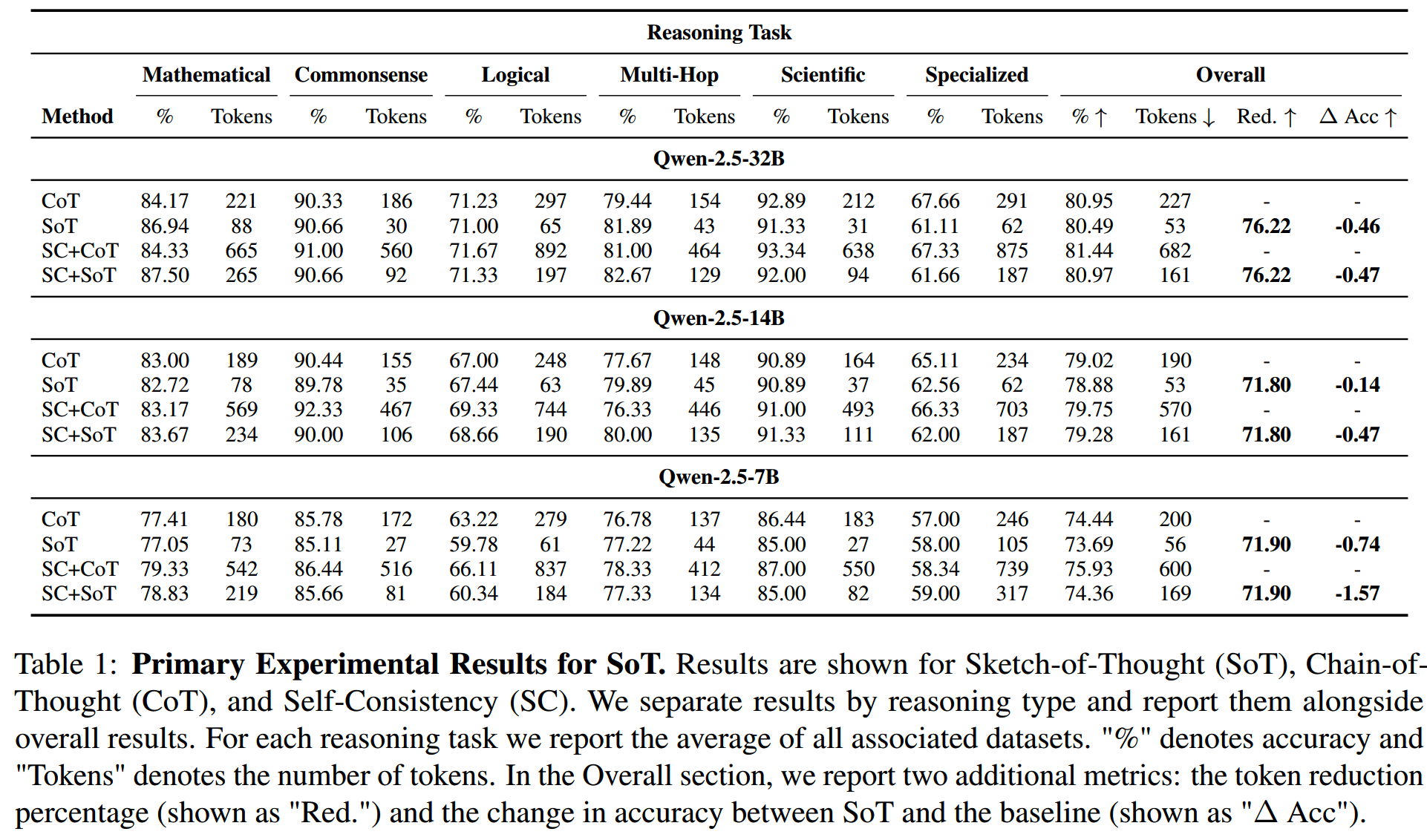
InftyThink
一句话,InftyThink把线性连续的reasoning过程,转化成包含reasoning summary的迭代过程。
看下面这张图就大概明白了:
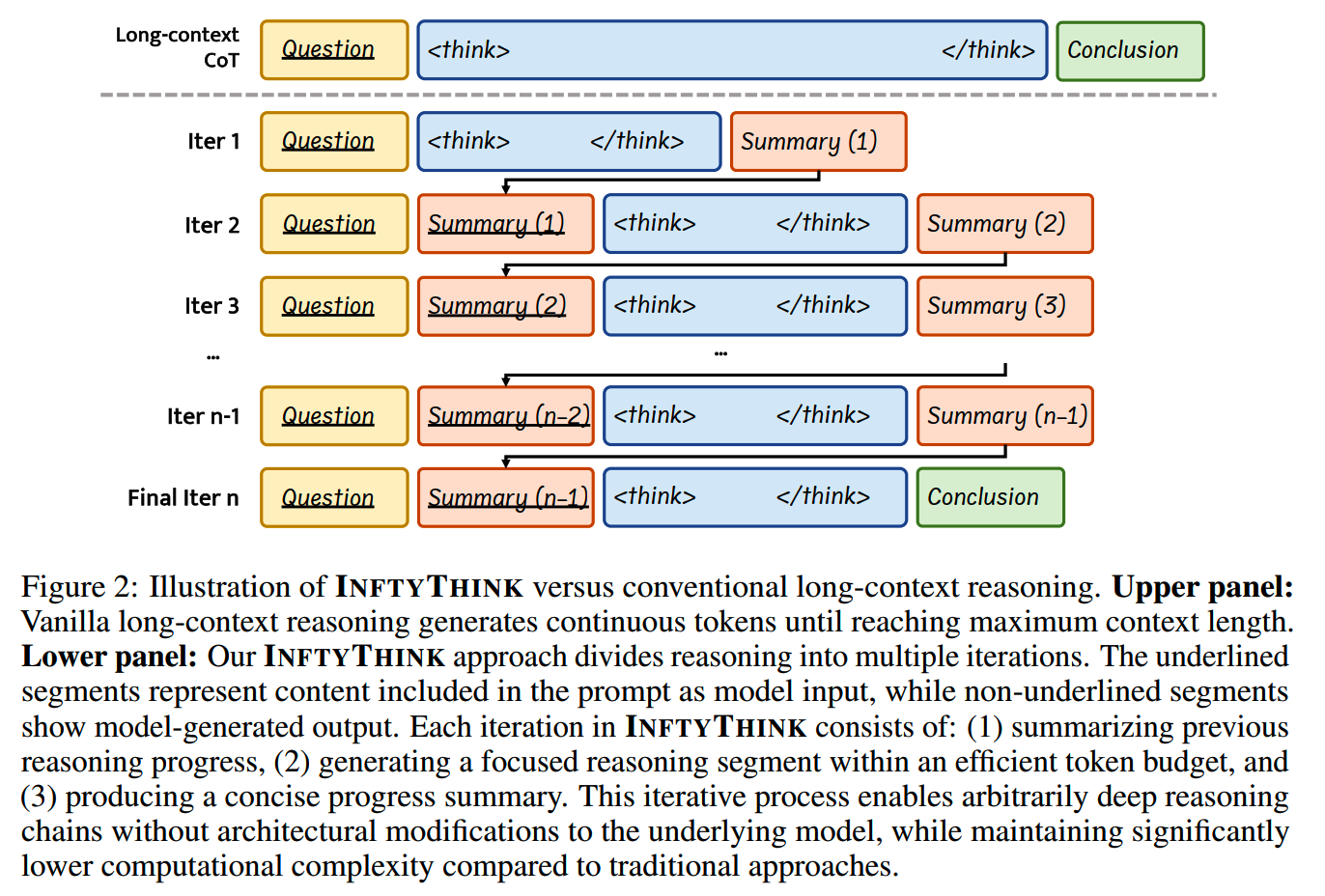
比如原来的CoT是把任务拆解成多个子问题,然后一个一个连续地输出结果。现在InftyThink不一次思考完所有问题,而是思考一部分之后,用一个summary prompt + LLM把前面的思考过程总结一下,替换原来是思考过程,然后再让模型在summary的基础上继续思考。
summary prompt:
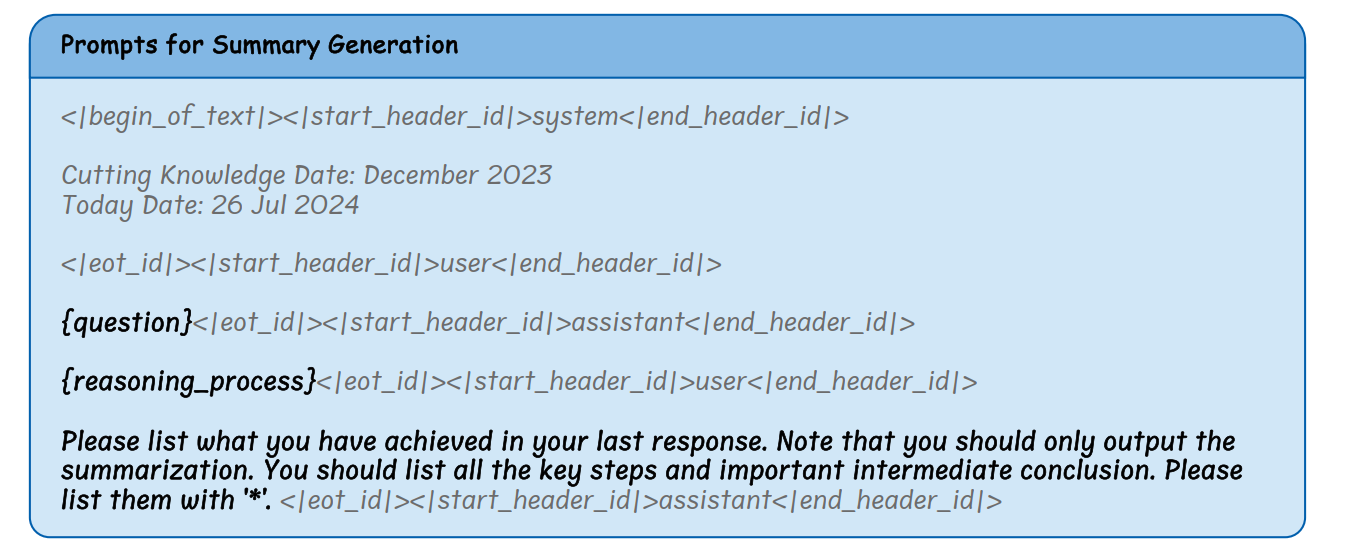
文中而用于生成summary的LLM是Llama-3.3-70B-Instruct。(一个问题,这里为什么不使用原模型来做summary呢?)
另外,怎么决定什么时候要做summary呢?首先定一个基础语义单位,比如是句子或者段落,模型生成的结果都会按这个切分。当一个完整的语义单位生成完之后,如果现有的思考长度超过某个阈值(比如4k token),那就会触发summary。
由于summary比原思考过程短,且迭代越多短得越多,因此可以减少整个思考过程的长度。理论上InftyThink的做法支持无限长度的生成。
由于思考的方式变了,因此模型需要重新训练,来适应这种一段一段summary + 生成的方式。训练用的数据从CoT数据改造的包含summary的数据:
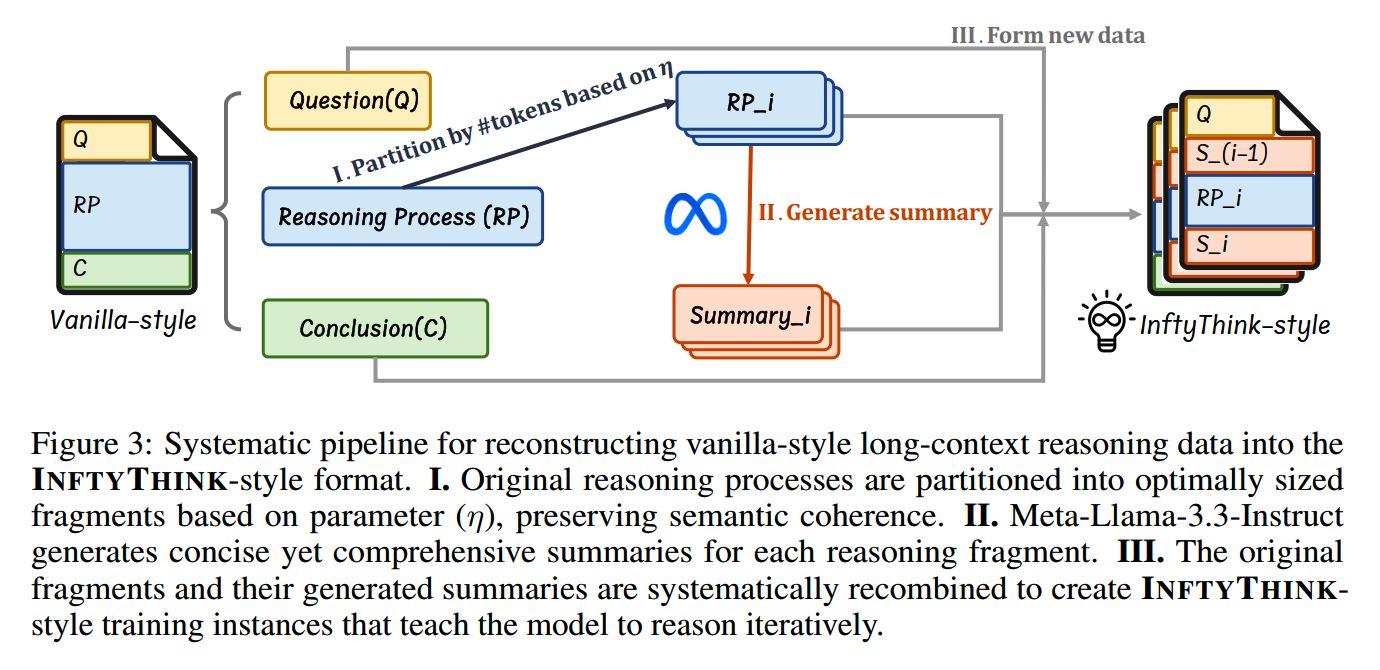
效果上,InftyThink在OpenR1-Math的准确率有所提升,整个过程的计算程度也更短:
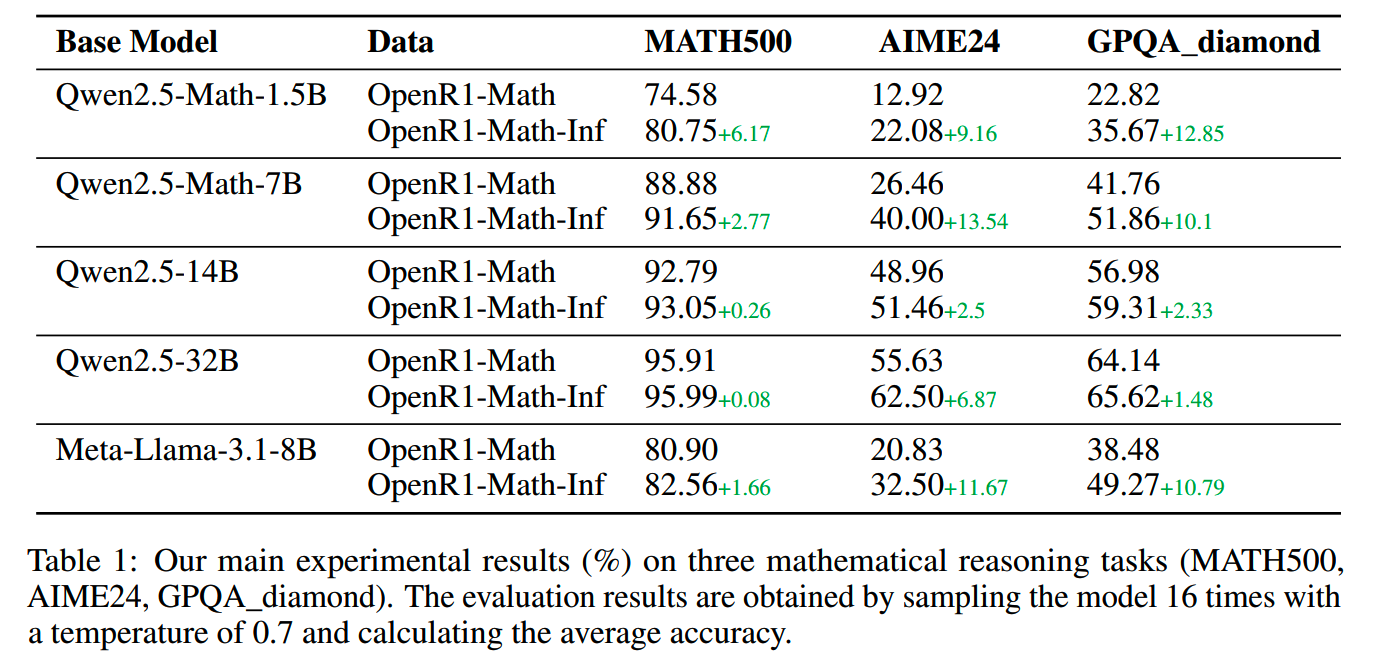
小结
prompt engineer的含金量还在提升,模型越强prompt效果越好。赶紧都从过往的人类思维研究里找一些方法放到prompt里。
博客:http://www.linsight.cn/
知乎:Linsight
微信公众号:Linsight
博主微信号(添加请注明来意):
【推荐文章】
- MoE:
MoE模型的前世今生
DeepSeek-V2和MLA
昆仑万维-SkyworkMoE
成本10w刀的JetMoE
MoE的top-p
routing
对MoE模型的一些观察
从dense到MoE -- sparse
upcycling
MoE路由--expert choice
routing
- 端侧模型:
苹果智能系统模型--AFM
MiniCPM
适合移动设备的语言模型--MobileLLM
phi系列模型
Gemma2
苹果的OpenELM
bilibili的index-1.9B
- 预训练:
DeepSeek-V3细节探索
代码大模型(一)--业界现状
代码大模型(二)--OpenCoder
LLM高效预训练(一)
LLM高效预训练(二)
Llama3.1--预训练要点一览
Qwen2技术报告
Yi技术报告-划重点看细节
InternLM系列模型
GLM4报告的一些技术点
从Yuan2.0到Yuan2.0-M32
从loss视角理解大模型涌现能力
- 数据:
训练数据合成(一)
训练数据合成(二)
训练数据合成(三)
LLM预训练数据策略(一)
预训练数据处理--长度分解
- 长上下文:
Qwen2.5-1M技术解析
LLM长上下文的问题
解锁大模型长上下文能力
大模型推理窗口-从有限到无限大
prompt压缩(一)
prompt压缩(二)
- 推理加速:
大模型推理加速-投机解码
大模型推理加速-MEDUSA
- 对齐:
深度求索DeepSeek-R1详解
Llama3.1--post-training要点一览
模型平均 -- model
soup
大模型偏好对齐-DPO
大模型偏好对齐-ODPO
大模型偏好对齐-simPO
大模型偏好对齐-IPO
- Transformer:
理解Attention:从起源到MHA,MQA和GQA
LLM的重复生成和ICL
transformer中normalization的二三事
从代码实现看normalization-到底做了什么
稀疏注意力计算:sliding
window attention
理解LLM位置编码:RoPE
RoPE的远距离衰减
LLM水印
- 项目应用:
一个模型支持智能助手系统
- CV:
CV入门--关于Vision
Transformer
CV入门--无监督学习
- 多模态:
多模态入门(一)--CLIP
多模态入门(二)--Flamingo,LLaVA系列和BLIP系列
多模态入门(三)--MiniGPT4,DeepSeekVL,InternVL系列和QwenVL系列
多模态入门(四)--CogVLM,VILA,MM1,MM1.5和Pixtral-12B
多模态入门(五)--InternVL系列
小米的移动UI多模态模型--MobileVLM
DeepSeek-VL2的细节
- 大模型算法题:
(1)、 (2)、 (3)、 (4)、 (5)、 (6)、 (7)、 (8)、 (9)
Reference
【1】Token-Budget-Aware LLM Reasoning
【2】Chain of Draft: Thinking Faster by Writing Less
【3】Sketch-of-Thought: Efficient LLM Reasoning with Adaptive
Cognitive-Inspired Sketching
【4】InftyThink: Breaking the Length Limits of Long-Context Reasoning in
Large Language Models