代码大模型(一)--业界现状
【本文已在同名 微信公众号 / 知乎 / 个人博客linsight.cn 上线】
借助代码大模型进行开发几乎已经是每个开发人员的日常了。代码模型是如何具备强大的代码能力的呢?今天来学习下业界几个比较热门的代码模型。
评测指标
在了解代码模型的训练之前,先了解下目前常用的一些评测指标。
HumanEval
HumanEval由OpenAI在《Evaluating Large Language Models Trained on Code》提出,共包含164个python编程问题。为了尽量避免和模型的训练数据重复(目前代码模型的训练数据几乎包含了网络上能找到的所有代码数据),这些编程问题是人工专门编写的。
HumanEval原始数据中,每个问题包含以下字段:
- task_id:每个问题的id,如“HumanEval/0”
- prompt:编程问题的主体,形如
from typing import List
def has_close_elements(numbers: List[float], threshold: float) -> bool:
""" Check if in given list of numbers, are any two numbers closer to each other than
given threshold.
>>> has_close_elements([1.0, 2.0, 3.0], 0.5)
False
>>> has_close_elements([1.0, 2.8, 3.0, 4.0, 5.0, 2.0], 0.3)
True
"""
- canonical_solution:参考答案,形如
for idx, elem in enumerate(numbers):
for idx2, elem2 in enumerate(numbers):
if idx != idx2:
= abs(elem - elem2)
distance if distance < threshold:
return True
return False
- test:单元测试的样例,HumanEval平均每个问题有 7.7 个测试样例
= {
METADATA 'author': 'jt',
'dataset': 'test'
}
def check(candidate):
assert candidate([1.0, 2.0, 3.9, 4.0, 5.0, 2.2], 0.3) == True
assert candidate([1.0, 2.0, 3.9, 4.0, 5.0, 2.2], 0.05) == False
assert candidate([1.0, 2.0, 5.9, 4.0, 5.0], 0.95) == True
assert candidate([1.0, 2.0, 5.9, 4.0, 5.0], 0.8) == False
assert candidate([1.0, 2.0, 3.0, 4.0, 5.0, 2.0], 0.1) == True
assert candidate([1.1, 2.2, 3.1, 4.1, 5.1], 1.0) == True
assert candidate([1.1, 2.2, 3.1, 4.1, 5.1], 0.5) == False
- entry_point:代码运行的入口,用于评测
论文中,使用的是pass@k的评测指标,即模型会多次进行生成采样,计算通过评测的期望值。而在OpenCompass中,默认是使用pass@1,即只生成一次,并在对应的评测样例上进行测试。
OpenCompass在生成的时候,默认会加上个prompt:“Complete the following python code:”,即模型的输入是
Complete the following python code:from typing import List
def has_close_elements(numbers: List[float], threshold: float) -> bool:
""" Check if in given list of numbers, are any two numbers closer to each other than
given threshold.
>>> has_close_elements([1.0, 2.0, 3.0], 0.5)
False
>>> has_close_elements([1.0, 2.8, 3.0, 4.0, 5.0, 2.0], 0.3)
True
"""
OpenCompass调用的是OpenAI的evaluate_functional_correctness接口进行评测的,输入就是模型生成的代码以及测试样例。
MBPP
MBPP = Mostly Basic Programming Problems,而OpenCompass上最常用就是MBPP-python。
MBPP由谷歌在《Program Synthesis with Large Language Models》中引入。每个问题也是由人工编写的,每个问题包含3个测试用例。
MBPP中,有 58% 的问题是数学相关的(如计算球体体积),43% 涉及列表处理,19% 需要字符串处理,9% 涉及整数序列,2% 涉及其他数据结构。参考解决方案的代码行数平均为 6.8 行,中位数为 5 行,最多为 50 行,自然语言描述通常简短,一般为一句话。
原始数据中,每个问题包含以下字段: - task_id:每个问题的id
- text:问题的文字描述,如:“Write a function to find the maximum
difference between available pairs in the given tuple list.”
- code:参考答案,如
= 3
R = 3
C def min_cost(cost, m, n):
= [[0 for x in range(C)] for x in range(R)]
tc 0][0] = cost[0][0]
tc[for i in range(1, m+1):
0] = tc[i-1][0] + cost[i][0]
tc[i][for j in range(1, n+1):
0][j] = tc[0][j-1] + cost[0][j]
tc[for i in range(1, m+1):
for j in range(1, n+1):
= min(tc[i-1][j-1], tc[i-1][j], tc[i][j-1]) + cost[i][j]
tc[i][j] return tc[m][n]
- test_list:测试样例,如
'assert min_cost([[1, 2, 3], [4, 8, 2], [1, 5, 3]], 2, 2) == 8', 'assert min_cost([[2, 3, 4], [5, 9, 3], [2, 6, 4]], 2, 2) == 12', 'assert min_cost([[3, 4, 5], [6, 10, 4], [3, 7, 5]], 2, 2) == 16'] [
- challenge_test_list:比较难的测试样例,只有部分问题有,如
'assert remove_Occ("hellolloll","l") == "helollol"', 'assert remove_Occ("","l") == ""'] [
test_setup_code:关于评测条件的一些设置,只有极少量问题有,如
= Node(1)
root = Node(2)
root.left = Node(3)
root.right = Node(4)
root.left.left = Node(5)
root.left.right = Node(8)
root.left.left.left = Node(1)
root1 = Node(2)
root1.left = Node(3)
root1.right = Node(4)
root1.left.left = Node(5)
root1.left.right = Node(6)
root1.right.left = Node(7)
root1.left.left.left = Node(1)
root2 = Node(2)
root2.left = Node(3)
root2.right = Node(4)
root2.left.left = Node(5)
root2.left.right = Node(7) root2.left.left.left
OpenCompass在评测时,会使用其中500条,并加上few-shot样本,这些样本是固定的,也是来自MBPP数据集。prompt如下:
and here is your task: Write a function to find the similar elements from the given two tuple lists. Your code should pass these tests:
You are an expert Python programmer,
assert similar_elements((3, 4, 5, 6),(5, 7, 4, 10)) == (4, 5)
assert similar_elements((1, 2, 3, 4),(5, 4, 3, 7)) == (3, 4)
assert similar_elements((11, 12, 14, 13),(17, 15, 14, 13)) == (13, 14)
[BEGIN]'def similar_elements(test_tup1, test_tup2):
res = tuple(set(test_tup1) & set(test_tup2))
return (res)'
[DONE]
You are an expert Python programmer, and here is your task: Write a python function to identify non-prime numbers. Your code should pass these tests:
assert is_not_prime(2) == False
assert is_not_prime(10) == True
assert is_not_prime(35) == True
[BEGIN]'import math
def is_not_prime(n):
= False
result for i in range(2,int(math.sqrt(n)) + 1):
if n % i == 0:
= True
result return result'
[DONE]
You are an expert Python programmer, and here is your task: Write a function to find the largest integers from a given list of numbers using heap queue algorithm. Your code should pass these tests:
assert heap_queue_largest( [25, 35, 22, 85, 14, 65, 75, 22, 58],3)==[85, 75, 65]
assert heap_queue_largest( [25, 35, 22, 85, 14, 65, 75, 22, 58],2)==[85, 75]
assert heap_queue_largest( [25, 35, 22, 85, 14, 65, 75, 22, 58],5)==[85, 75, 65, 58, 35]
[BEGIN]'import heapq as hq
def heap_queue_largest(nums,n):
= hq.nlargest(n, nums)
largest_nums return largest_nums'
[DONE]
You are an expert Python programmer, and here is your task: Write a python function to remove first and last occurrence of a given character from the string. Your code should pass these tests:
assert remove_Occ("hello","l") == "heo"
assert remove_Occ("abcda","a") == "bcd"
assert remove_Occ("PHP","P") == "H"
[BEGIN]
最后一个[DONE]后面的才是需要模型解决的问题,前面都是固定的prompt样本。
和HumanEval略有不同,MBPP评测的时候模型可以看到将用于评测的case。
其他评测
HumanEval和MBPP都属于代码生成评测的数据集,并且都是python的。除了这两个,代码生成的评测最近比较常见的还有BigCodeBench(BigCodeBench-Instruct),HumanEval的增强版本EvalPlus等。
另外还有评测代码补全的如RepoBench,以及在FIM(待会讲)中提出的各种Infilling benchmark。
Code Fixing也有SWE-bench和Aider等评测数据。
不过最近优先关注HumanEval和MBPP的评测得分,所以其他能力以后再展开。
FIM
FIM = fill-in-the-middle,是一种模型训练方式,这里参考OpenAI的《Efficient Training of Language Models to Fill in the Middle》来介绍一下。
首先需要了解为什么需要FIM的训练方式。目前我们知道GPT模型相比Bert类模型,有更高的训练效率;而从左到右自由生成的方式也使得GPT模型能够应用在更多场景,上限更高。但是传统的left-to-right的训练方式也有限制:如在代码补全的场景,需要模型同时兼顾上文和下文,对中间部分的内容进行补全,这种情况下left-to-right的训练方式就无法提供有效的信息,因为看不见下文。
为了解决这个问题,可以对模型的输入数据做一个transformation:把原本顺序正常的文档,切分成三部分,即prefix、middle和suffix,并把middle部分放到最后面。
document -> (prefix; middle; suffix) -> (prefix; suffix; middle)
训练的时候,模型需要根据给定的上文prefix和下文suffix,来生成中间的部分。
FIM效果
为了让模型同时具备正常的从左到右的生成能力,需要使用left-to-right和FIM两个方式混合的数据,FIM数据的比例成为FIM rate。
在实际训练模型中,OpenAI使用FIM rate = 0.5,即训练数据中一半进行了这种切分和转换,另一半保持正常的从左到右顺序。
实验中发现,混入FIM数据之后,模型在正常left-to-right的能力上基本上没有收到任何损害,如下图
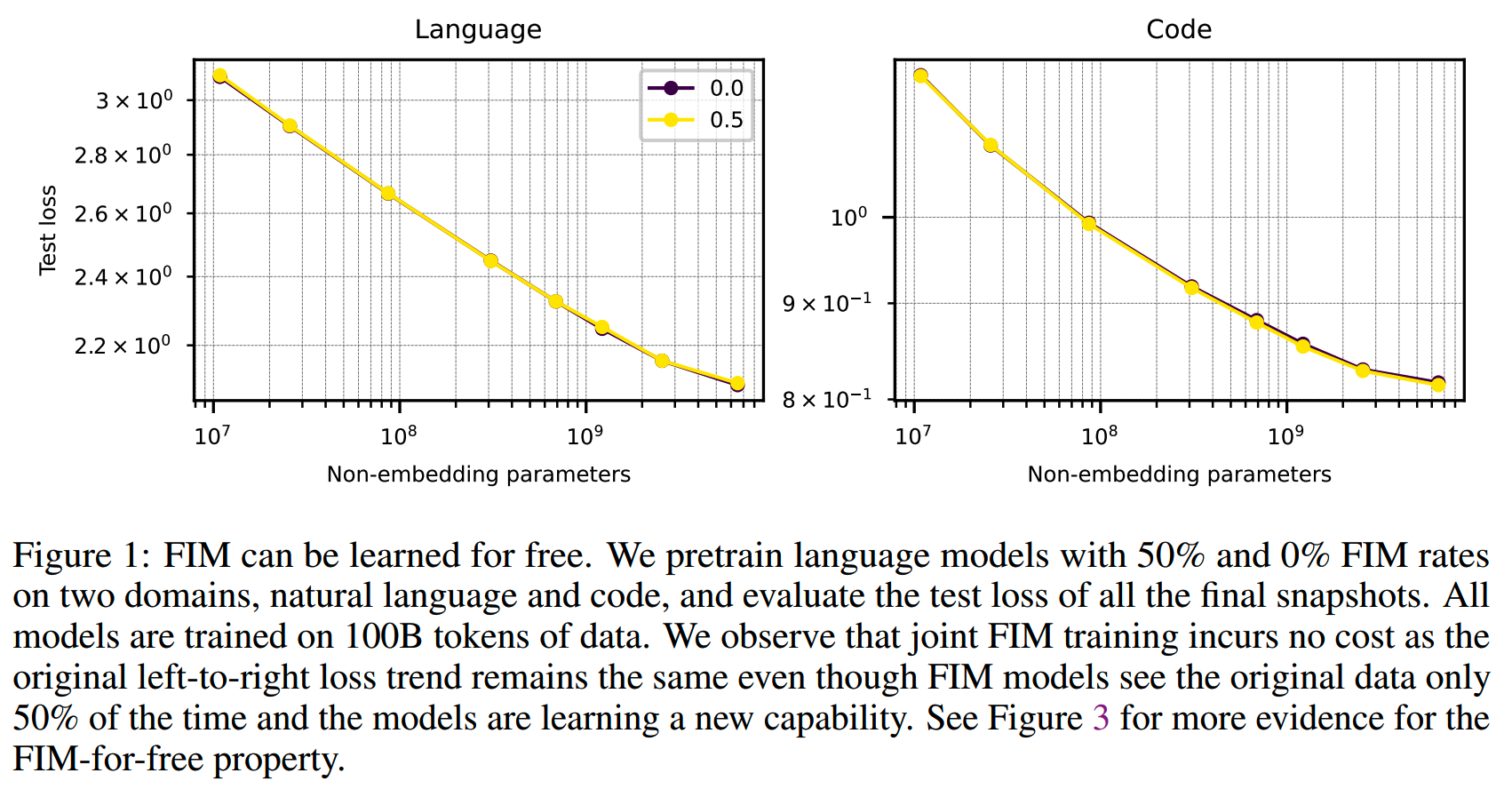
相当于模型没有付出任何代价就多学到了FIM的能力,OpenAI称之为FIM-for-free property。
通常的PPL测试不能看出FIM带来的收益,为了评测模型FIM,OpenAI专门构建一个infilling benchmark。infilling benchmark中的数据来源于HumanEval,通过删除中间的部分代码,要求模型补全来检验FIM能力。下面是一个例子,绿色部分就是要模型补全的中间代码:
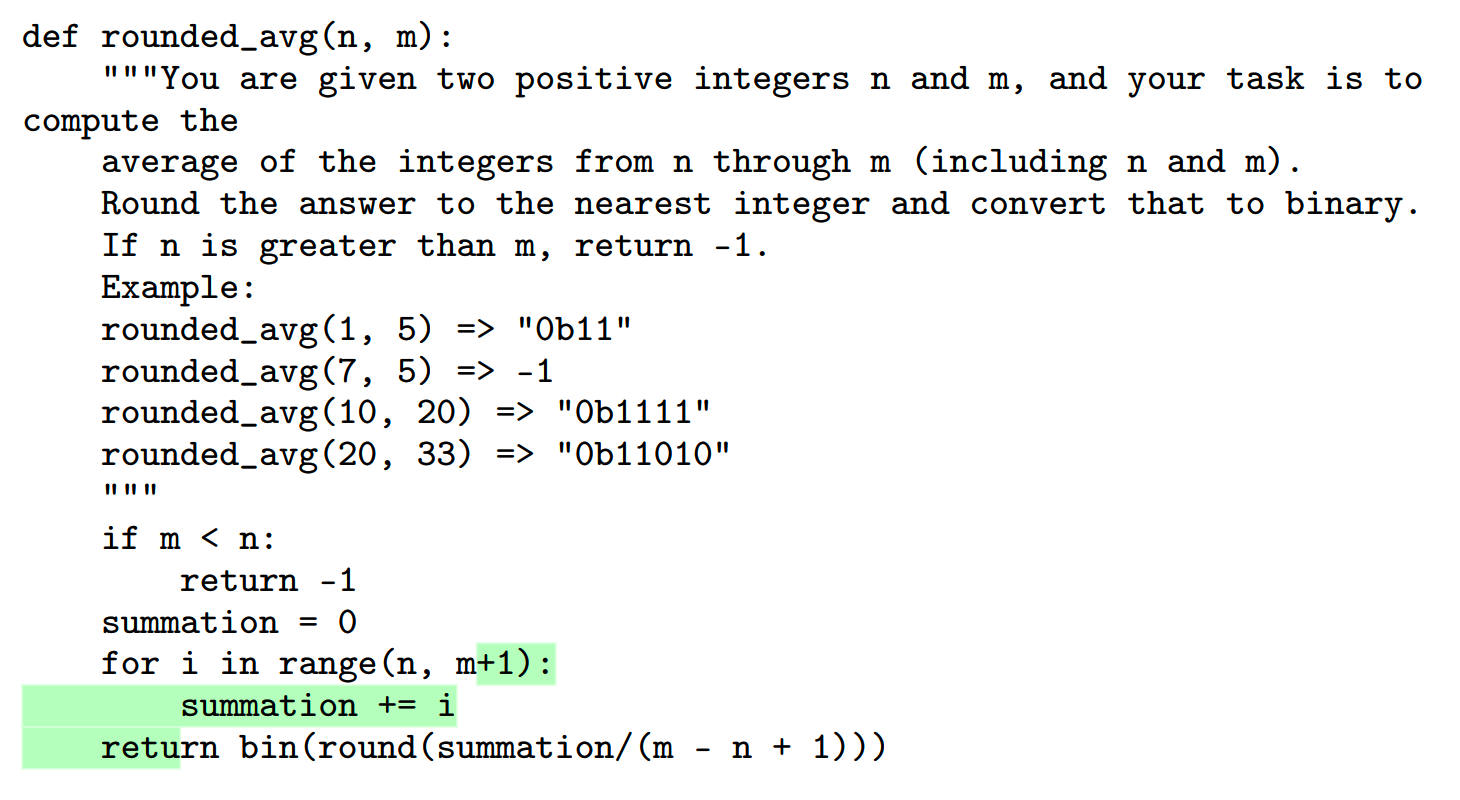
有无使用FIM数据的模型,在FIM测试上的对比如下:
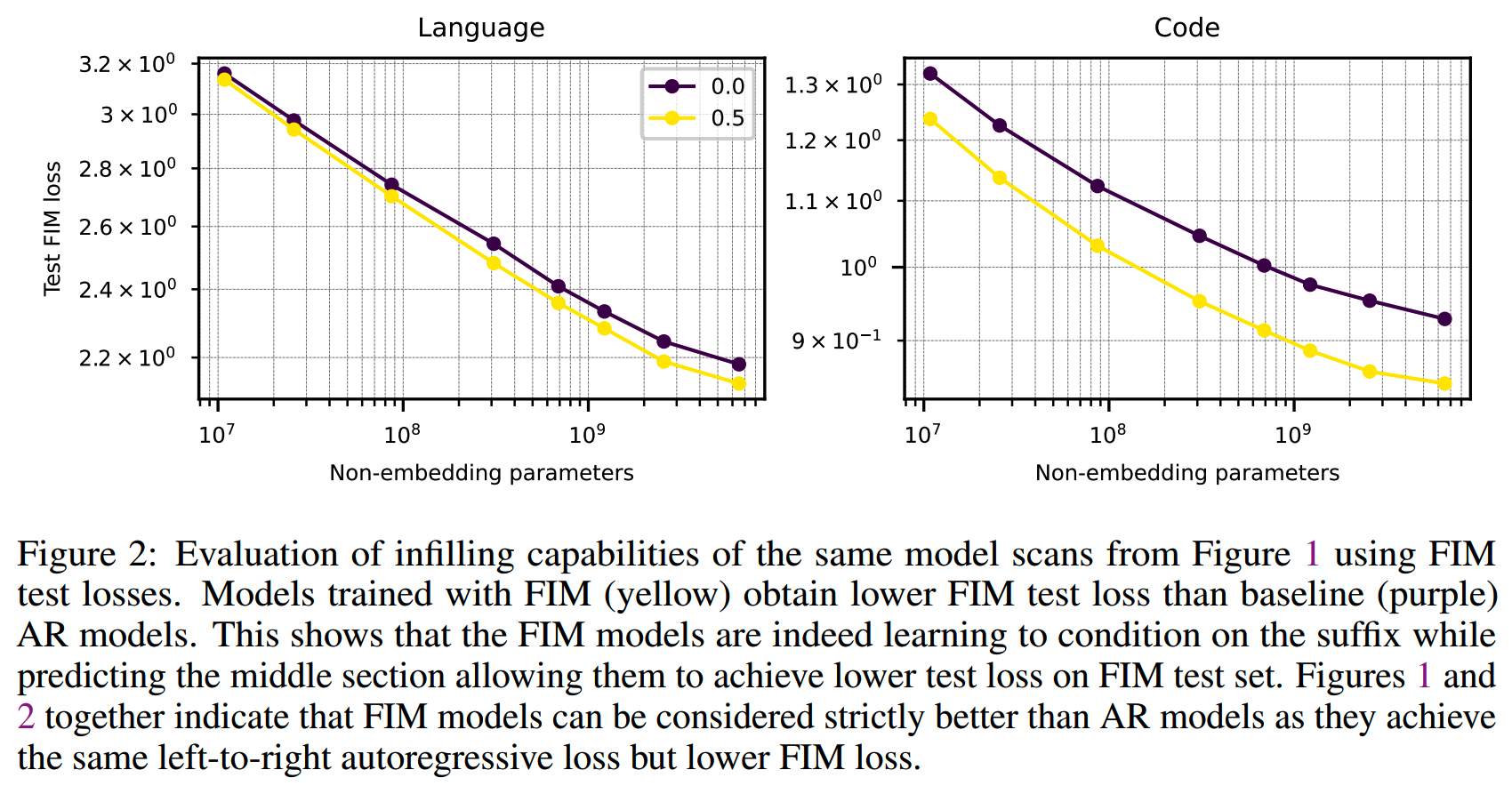
加入FIM明显地提升模型的infilling能力。
训练
前面简单介绍了FIM的做法,就是把数据切成前中后三部分,然后把中间部分挪到后面,要求模型补全。
更具体来说,为了让模型知道哪部分是prefix,哪部分是suffix,哪部分是middle,需要加入一些特殊token:
< PRE > ○ Enc(prefix) ○ < SUF > ○ Enc(suffix) ○ < MID > ○ Enc(middle)
○ 表示concat。< PRE >、< SUF >和< MID >就是用于标识数据位置的特殊token。
训练的时候,不止有middle部分的loss会bp,prefix和suffix也和left-to-right的数据一样会进行训练,这样FIM相比left-to-right并不会损失loss信号的量。
另外在每条训练数据最后还要记得加上< EOT >符号。
上面这种拼接方式,数据的顺序是Prefix、Suffix、Middle,简称为PSM。PSM是最符合直觉的一种拼接方式。而除了PSM,还可以使用SPM的拼接顺序。文中提到,SPM相比PSM有一个好处,那就是在推理的时候前面已经计算过的KV cache可以复用。(不过这里感觉有点奇怪,只要不把新生成的token拼接到Prefix部分,其实PSM的KV cache也是可以服用的)
那么PSM和SPM的效果如何?实验了SPM、PSM和二者一起训练,效果如下:
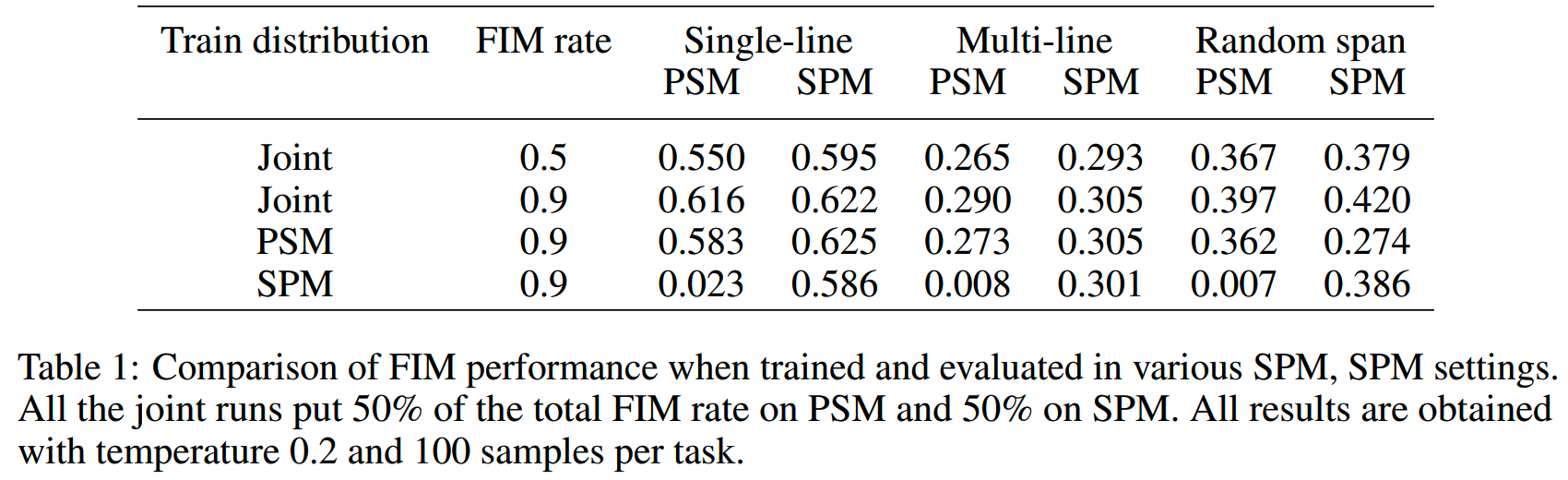
从结果上看,二者都用的效果是最好的。
FIM rate
FIM rate应该设置为多少合适?文中做了消融实验,对比FIM rate = (0, 0.25, 0.5, 0.75, 0.9, 1.0)的效果。结果如下:
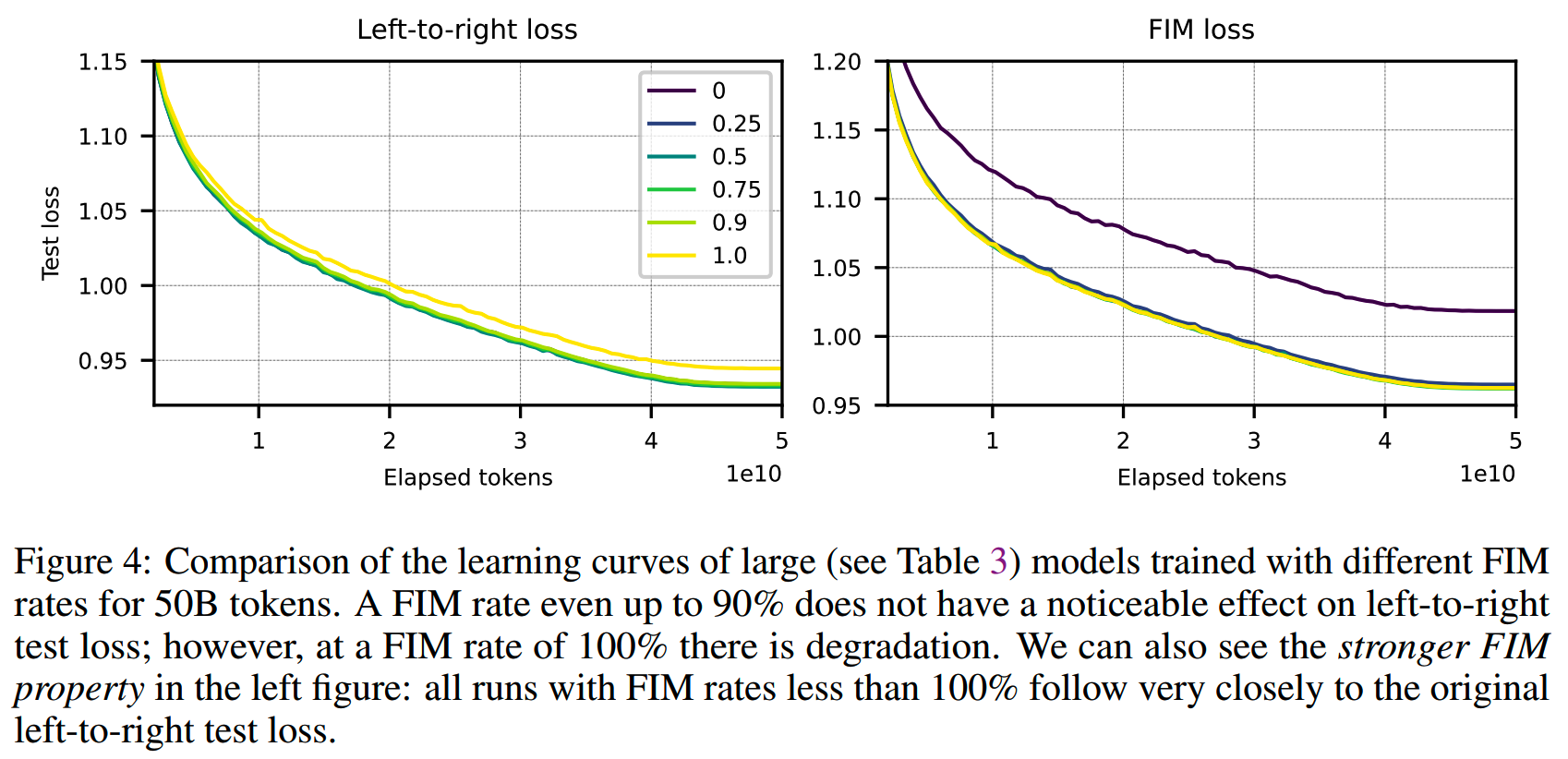
不同的FIM rate对left-to-right几乎没有什么影响(除了FIM rate = 1.0),而FIM loss则是只要使用FIM数据,就有明显提升。FIM rate = 0.5和0.9的效果差不多,最终使用的是0.5。
数据切分
前面说了数据要被切成三部分,那么具体怎么切?论文提供了三种粒度的对比,Line-level random span、Token-level random span、Character-level random span。三种粒度的切分都保证prefix、suffix、middle的期望长度都是总长度的1/3。结果如下:
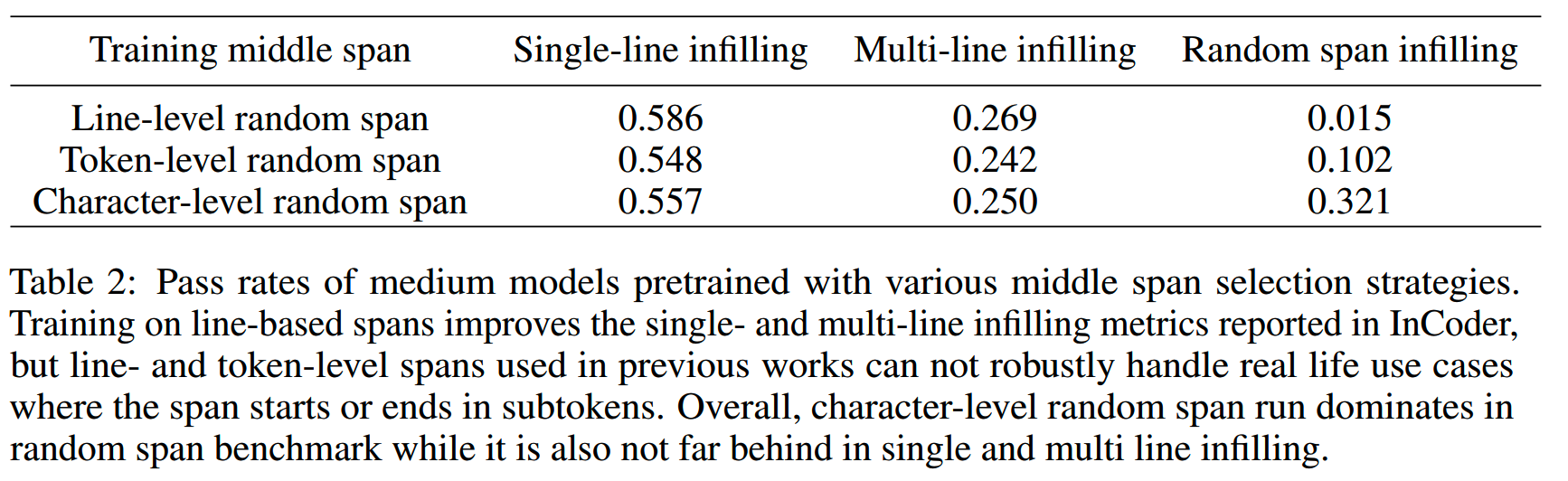
character level的整体效果最好。
Code Llama
接着来看下Code Llama的一些内容。
Code Llama是基于Llama 2开发的。Code Llama系列和Llama
2一样有4个规模,7B, 13B, 34B, 和
70B。各种规模都有3个版本(值得一提的是,Meta还专门做了个针对Python的版本):
- Code Llama: a foundational model for code generation tasks
- Code Llama - Python: specialized for Python
- Code Llama - Instruct: fine-tuned with human instructions and
self-instruct code synthesis data
各个版本的训练流程如下:
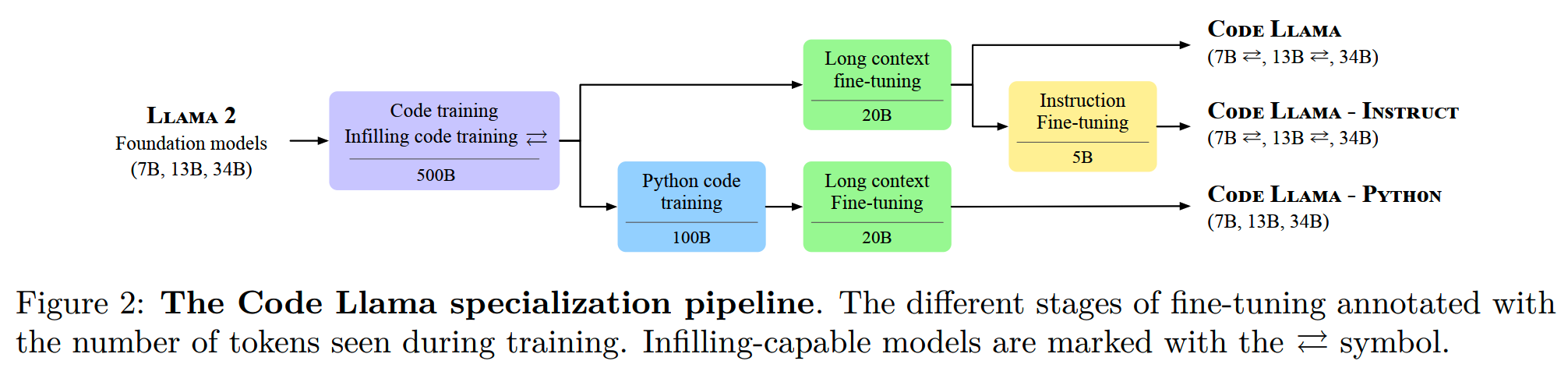
Code Llama效果也是不错,和其他模型的对比:
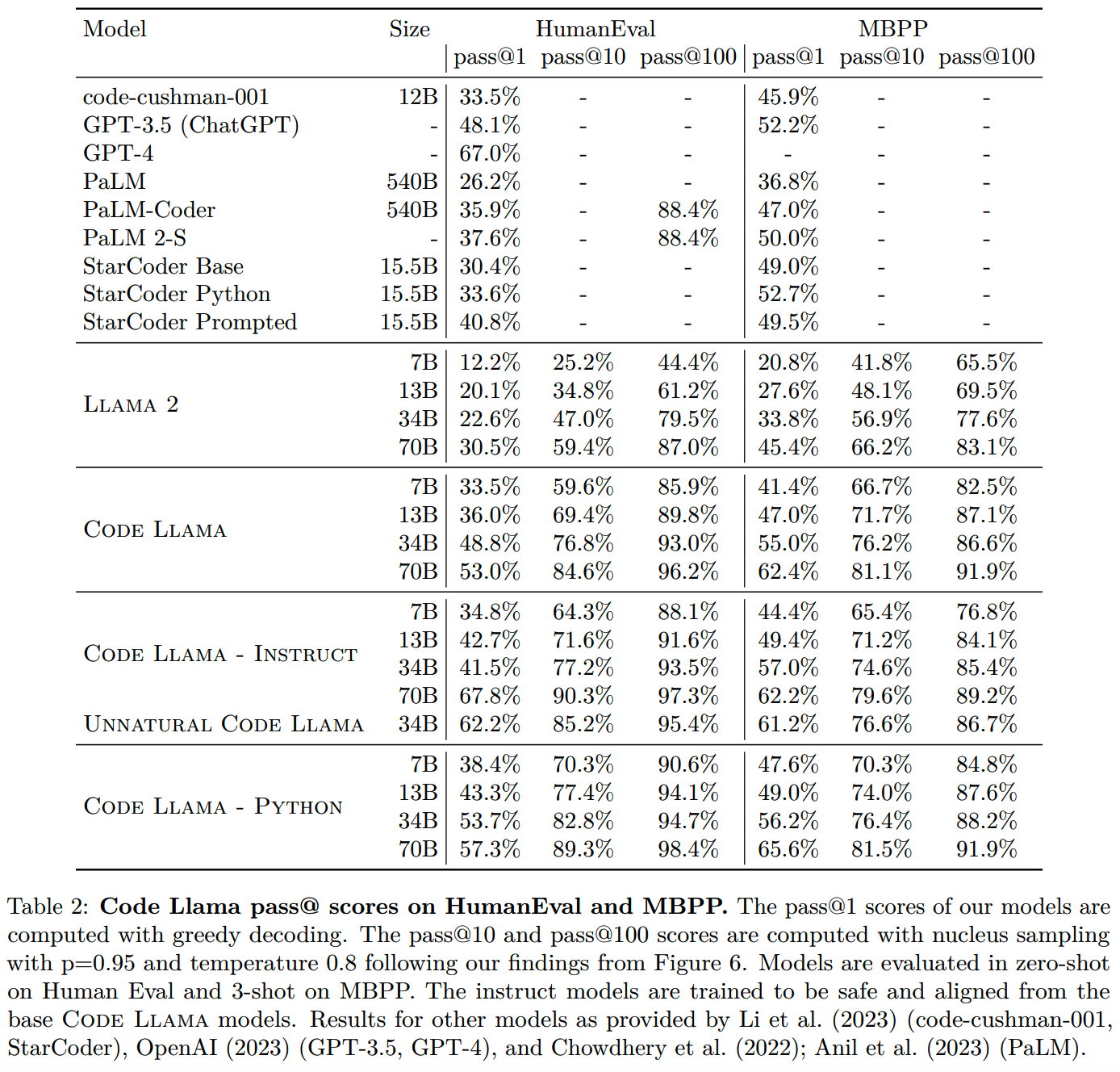
数据
Code Llama收集大约500B的训练数据,其中大部分是代码,另有少量从general数据集中抽取的代码相关数据,以及少量代码无关的数据:
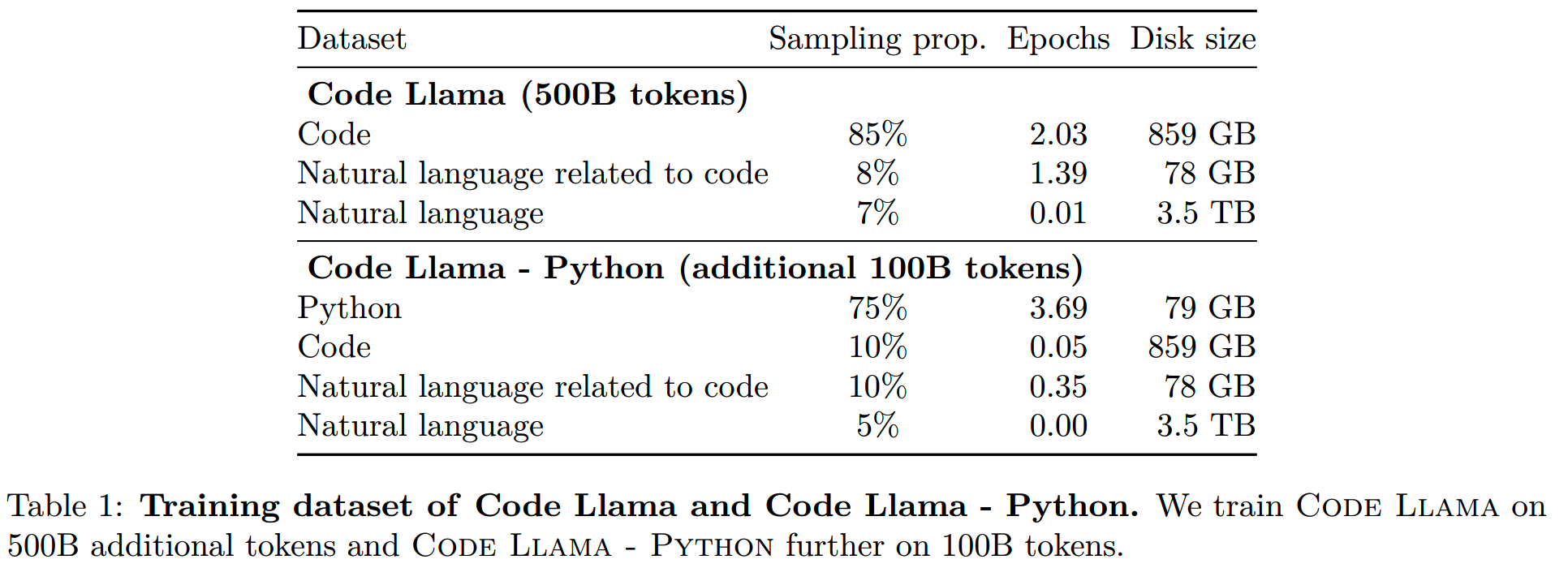
代码的数据都是训练了多个epoch。
而在微调的时候,则是使用了Llama 2 70B生成interview-style programming questions。所使用的prompt如下:
50 programming interview questions of easy and medium complexity. Provide questions on a
Prompt: [INST] Write range of subjects, and make sure no two questions are alike. Make sure the problems can be solved with a
diverse /INST]
single standalone Python function using standard libraries. [1. Write a function that finds the maximum depth of list nesting in a given list.
2. Given an integer array nums, rotate the array to the right by k steps, where k is non-negative.
3. Write a function that gets the musical pitch that is closest to a given frequency in Hz. A pitch should consist of a
# sign, and an octave number.
letter, possibly a 1. Write a function that removes any sequences of whitespace that are between numbers in an input string.
2. Write a function that counts the number of words in a string that have length n, where n is an input. The function
or spaces.
should ignore characters that aren’t letters, numbers, 1. Write a function that returns the longest palindromic substring in a given string.
2. Create a function that will find the length of the longest substring without repeating characters in a given string.
3. Write a function that reverses the diagits in a number.
4. Write a function that finds the valleys in a list of integers.
5. Write a python function that reverses every group of k words in a sentence.
去重之后得到了~52,000条问题。再把这些问题输入给Code Llama 7B生成单元测试样例和solution,prompt分别是:
Prompt:is to write 5 tests to check the correctness of a function that solves a programming
[INST] Your task
problem.and [/TESTS] tags.
The tests must be between [TESTS] "#Test case n:" on a separate line directly above each assert statement,
You must write the comment from 1 and increasing by one for each subsequent
where n represents the test case number, starting
test case.list.
Problem: Write a Python function to get the unique elements of a /INST]
[
[TESTS]# Test case 1:
assert get_unique_elements([]) == []
# Test case 2:
assert get_unique_elements([1]) == [1]
# Test case 3:
assert get_unique_elements([1, 2, 3, 2, 1]) == [1, 2, 3]
/TESTS]
[%%%question%%%
[INST] Problem: /INST] [
Prompt:is to write a Python function to solve a programming problem.
[INST] Your task and [/PYTHON] tags.
The Python code must be between [PYTHON] from which you can infere the function signature.
You are given one example test list.
Problem: Write a Python function to get the unique elements of a assert get_unique_elements([1, 2, 3, 2, 1]) == [1, 2, 3]
Test: /INST]
[
[PYTHON]def get_unique_elements(my_list):
return list(set(my_list))
/PYTHON]
[%%%question%%%
[INST] Problem: %%%test%%%
Test: /INST] [
最后把题目、单元测试样例和答案通过执行反馈的方式验证正确性。
Long Context Fine-Tuning
为了让模型具备长上下文的能力,应用了Long Context
Fine-Tuning(LCFT):
- 把RoPE的base frequency从10,000增大到1,000,000
- 把训练的最大长度从4k增大到100k
FIM训练
Code Llama训练的时候用到FIM的训练方式,同OpenAI的做法一样,FIM数据中一半使用PSM格式,另一半使用SPM格式,都是character level的split。不过这里使用各大的FIM rate:0.9。
FIM的评测效果:
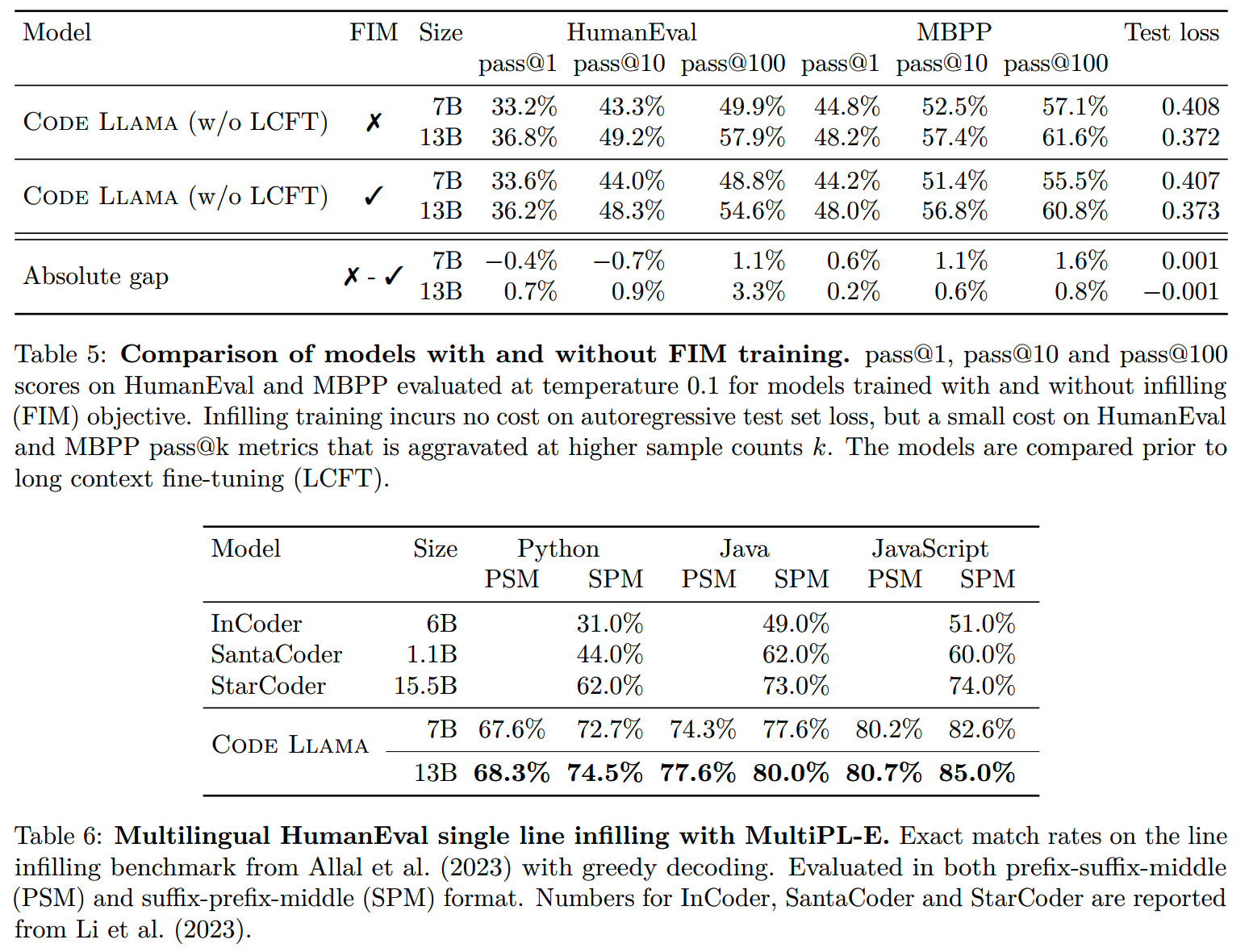
相比from scratch
Code Llama是以LLama 2为基础训练的,相比从零开始,使用代码数据进行训练的效果更好。如下图(b),sratch model相比Code Llama的loss明显更高:
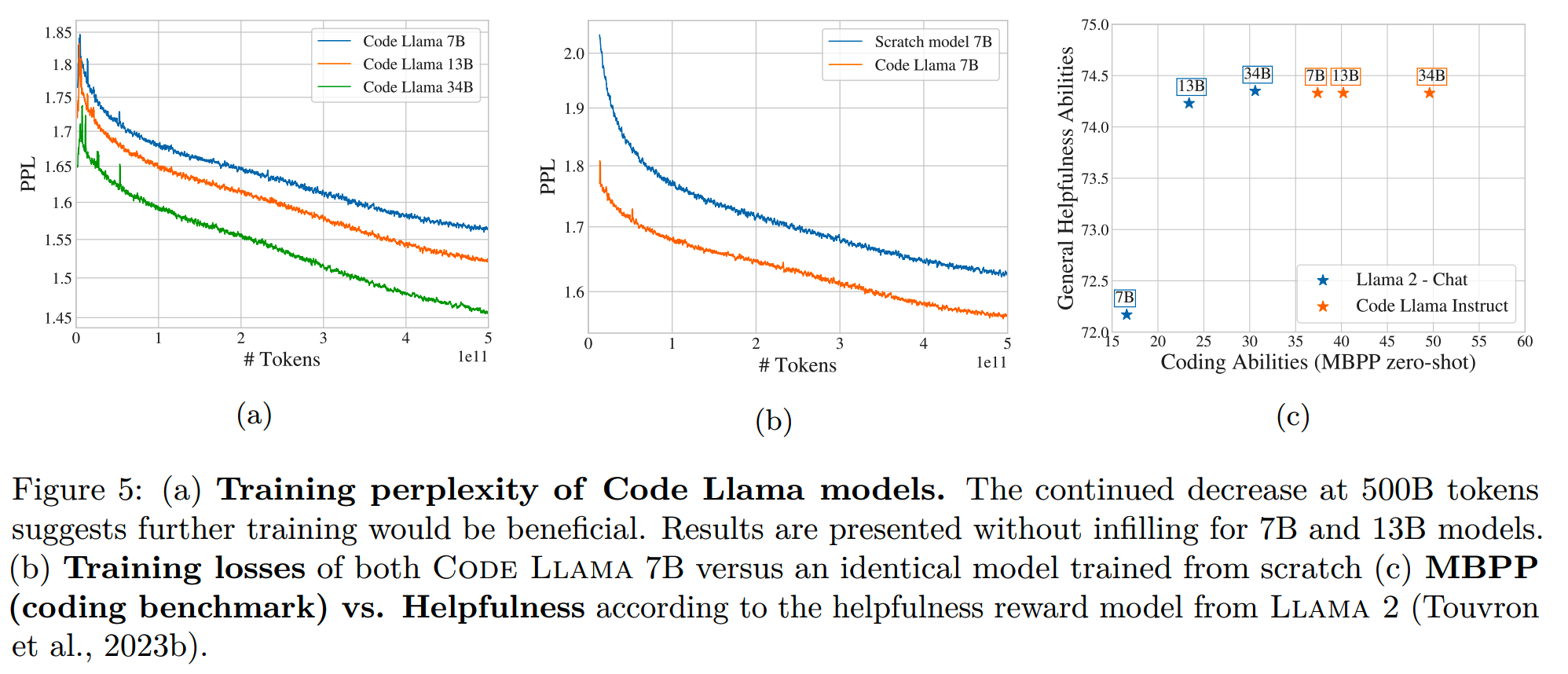
StarCoder 2
之前BigCode开源了StarCoder和Stack v1,几年继续打来StarCoder 2和Stack v2。v2版本的数据集是v1的4倍大,在这些数据集上,训练出了3B, 7B, 和 15B 的StarCoder 2。
数据
1、代码数据
基于Software Heritage的数据,涵盖619种语言。这些代码数据经过过滤(过滤网页自动生成的内容,恶意内容等)、去重、语言识别等处理。
2、Github Issues
包括issue的操作和内容。这些内容大都和代码的内容和开发相关,虽然不一定是代码数据,但是和代码有很大的相关性。
3、Pull Requests
这些不同分支的merge内容也能提供重要度high level信息。
4、Notebooks
包括Jupyter Notebooks,和Kaggle Notebooks,这些数据以外可能比较容易被忽略。
5、Documentation
来自各个包管理平台的文档,还有pdf文件,以及各种官方/教程网站。
6、其他高质量数据集
包含用于数学和编程的多个小数据集,如:
- GSM8K
- APPS
- Proofsteps
等
除了代码数据,还有必要加入一些自然语言的数据让模型学习,如 Stack Overflow、ArXiv、Wikipedia 和 OpenWebMath。
上面这些所有数据都会经过以下处理:
- simhash去重
- 个人信息Personally Identifiable Information (PII)删除
- Decontamination移除和评测集相关内容
- 恶意检测Malware Removal(有0.009%的数据在这步被移除)
最终各个规模模型的训练数据组成如下:
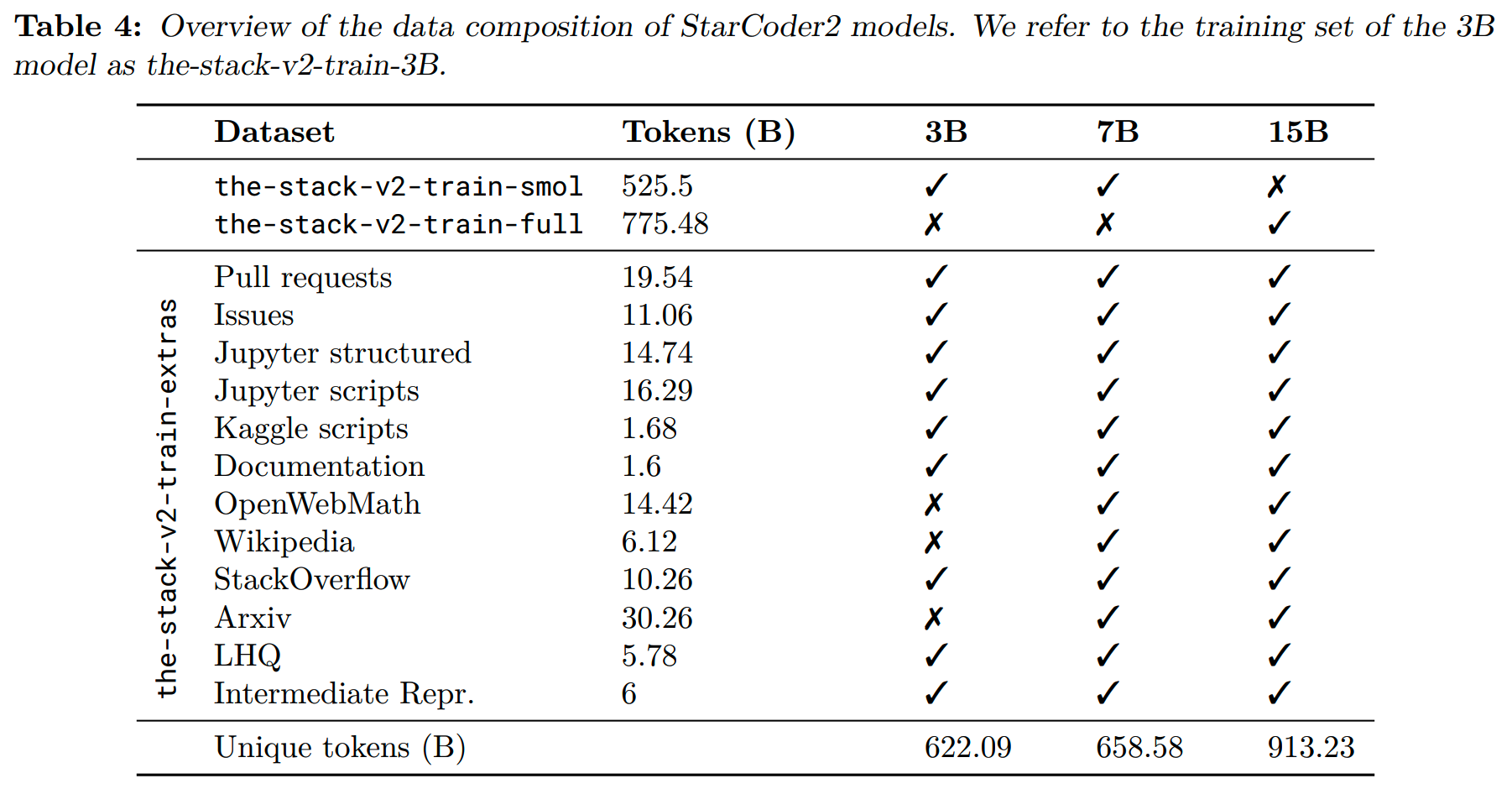
训练
收集的数据里有不同类型的数据,包括源码、notebook、issue、pull request,每种数据都有单独的拼接处理方式。比如源码会拼接成:
在没有meta data的情况下则是:
而pull request数据则是:
<pr>Title: title\nusername_0: description
<pr_status>opened
<repo_name>reponame
<pr_base>
<pr_file>filepath_1
<pr_base_code>file_content/changes_1
...<pr_file>filepath_N
<pr_base_code>file_content/changes_N
<pr_diff>
<pr_file>filepath_1
<pr_diff_hunk>diff_hunk_1
...<pr_diff_hunk>diff_hunk_K
...<pr_file>filepath_M
<pr_diff_hunk>diff_hunk_1
...<pr_diff_hunk>diff_hunk_J
因此增加了很多特殊token用于标识不同的内容:
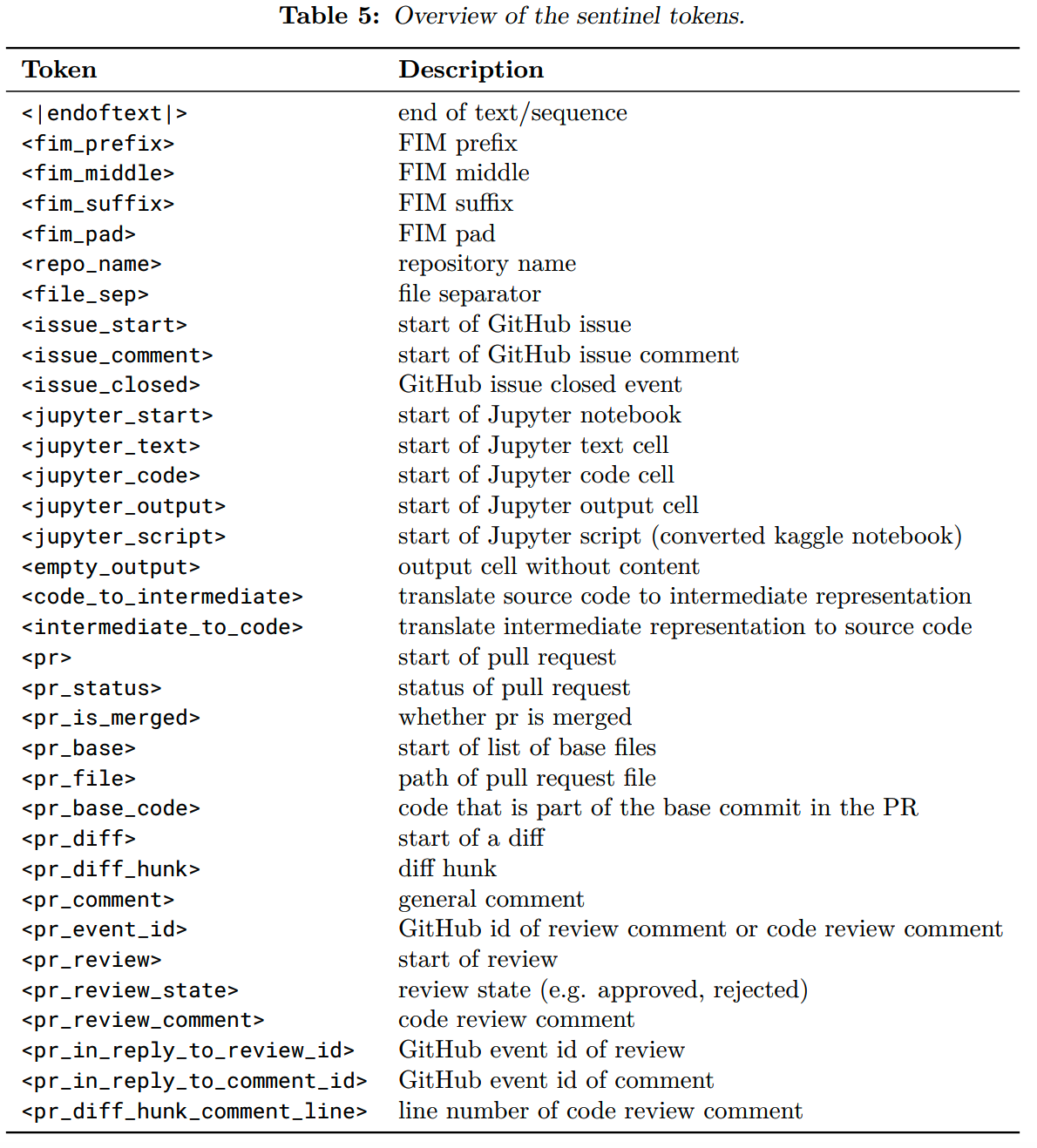
模型结构和在各自数据上的训练配置如下:
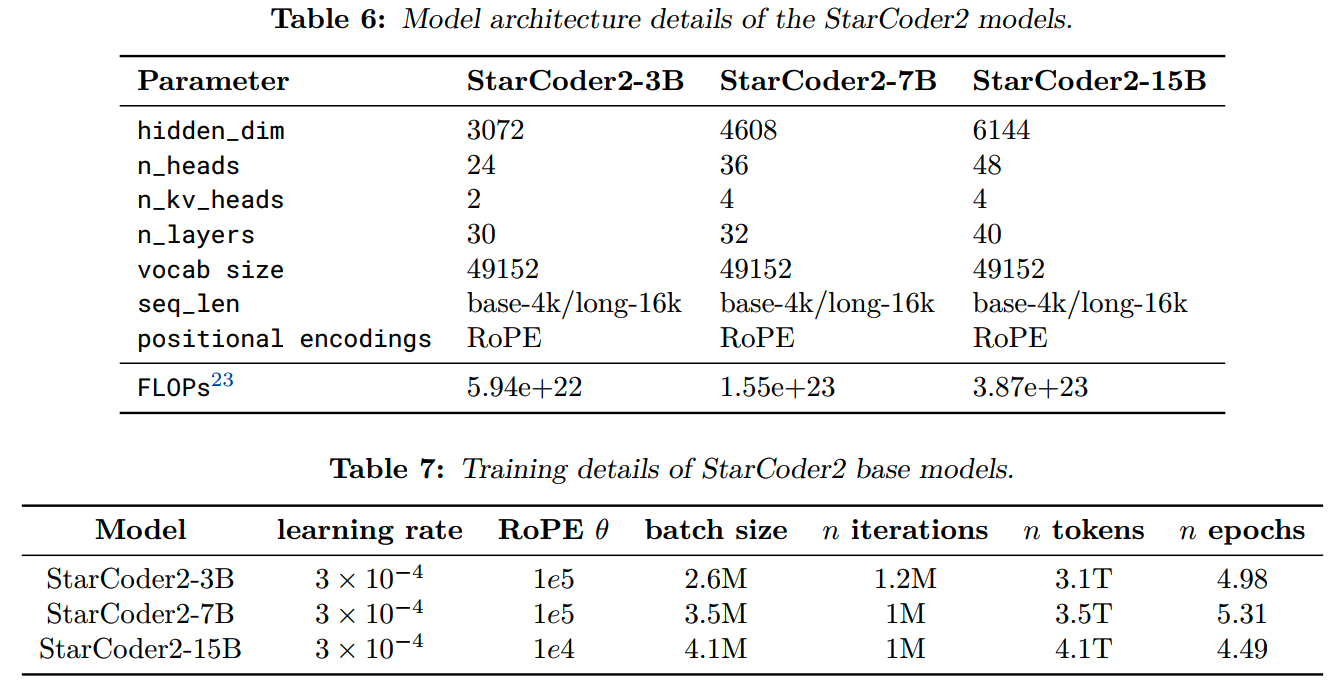
可以看到各个模型都在代码数据上训练的多个epoch。
DeepSeek-Coder-V2
DeepSeek-Coder-V2是在DeepSeek-V2(MoE模型)基础上训练的,对应DeepSeek-V2的两个规模:16B和236B,激活参数量分别为2.4B和21B。千亿模型的效果自然是很不错的:
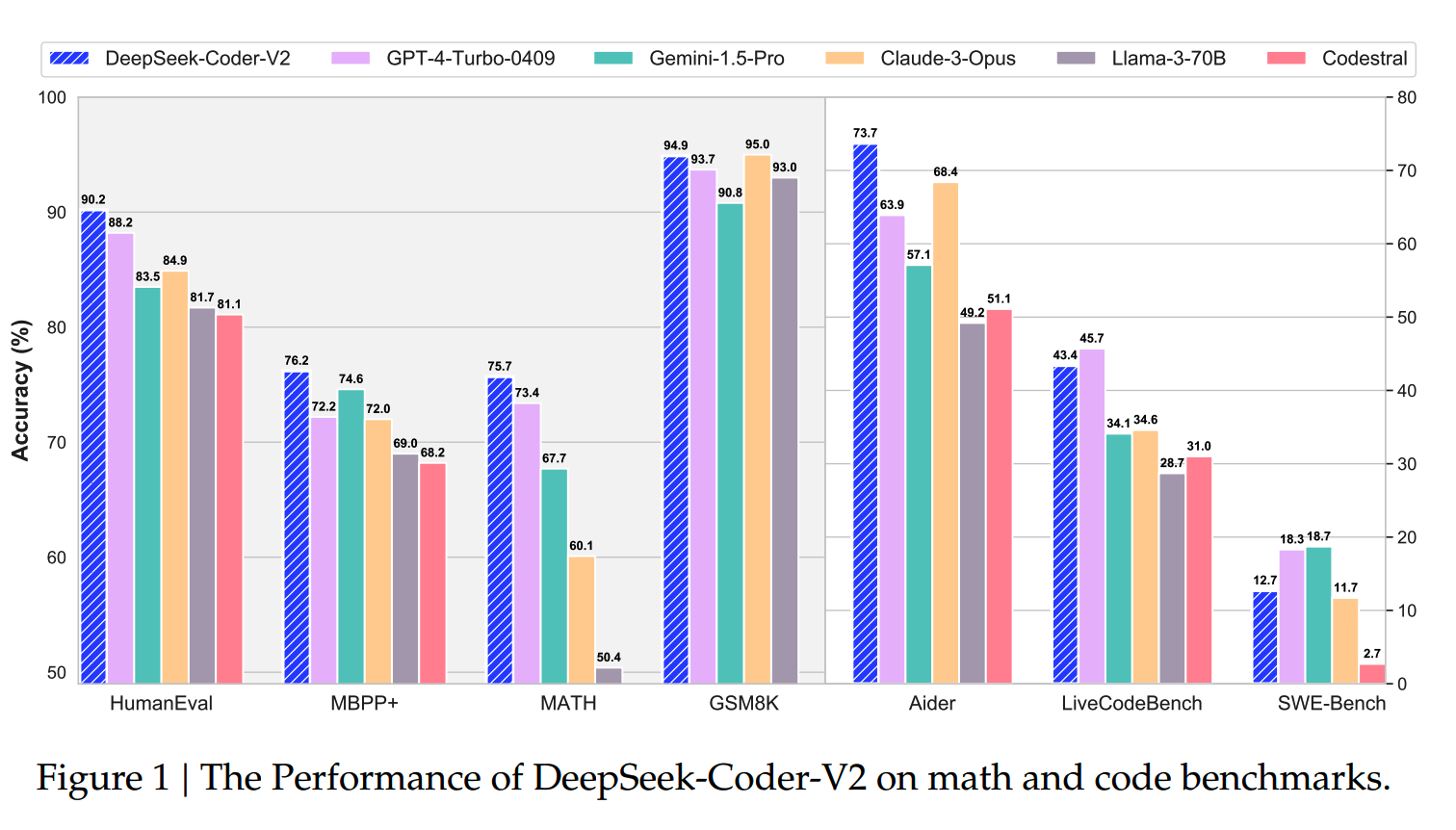
数据
DeepSeek-V2训练的4.2T的通用数据,DeepSeek-Coder-V2则是在这个基础,进一步训练6T token的数据。这6T数据里,有60%的source code,30%的数学语料,还有10%的自然语言数据,而重点自然就是代码数据和数学数据。
1、代码数据
原始代码数据来自2023年11月前的github数据。
文中给出了一些代码数据的筛选处理逻辑:
- 筛掉平均行长度 > 100 character或者最大行长度 > 1000
character的file
- 筛掉包含的alphabetic character比例 < 25%的file
- 除了XSLT语言,其他在开头100个character包含“< ?xml
version=”的文件会被删掉
- 对于HTML文件,要求visible
text的占比要大于20%,切至少100character
-
对于JSON和YAML这种通常是数据文件的类型,只保留长度为50到5000character的文件
这样清洗过后得到了821B包含338种语言的代码数据和185B的code-related数据(如markdown和issue)。
2、数学数据
对于code-related 和 math-related,follow the same pipeline as DeepSeekMath。
首先从一些相关网页,比如StackOverflow,PyTorch documentation或者StackExchange爬取数据。之后训练一个fasttext模型用于从网页数据里recall code-related 和 math-related 数据。
最终共收集到70B code-related数据和221B math-related数据。
为了检验这些数据的质量,用一个1B的模型在这些数据上进行训练。首先,使用 1T tokens 在新代码语料库上对 1B 模型进行预训练。然后,观察其在 HumanEval 和 MBPP 基准测试中的准确率变化。结果显示,在 HumanEval 基准测试中,准确率从 30.5% 提高到 36.0%,提高了 5.5%;在 MBPP 基准测试中,准确率从 44.6% 提高到 49.0%,提高了 4.4%。接着,进一步使用 2T tokens 对 1B 模型进行训练。再次观察在两个基准测试中的准确率,发现 HumanEval 和 MBPP 的分数分别上升到 37.2% 和 54.0%。

通过这些实验结果可以看出,新代码语料库在提高模型准确率方面表现更好,因此新代码语料库优于用于训练 DeepSeek-Coder-V1 的语料库。
训练
对于16B模型,使用了left-to-right和FIM两种训练方式,而对于236B模型,则没有使用FIM。
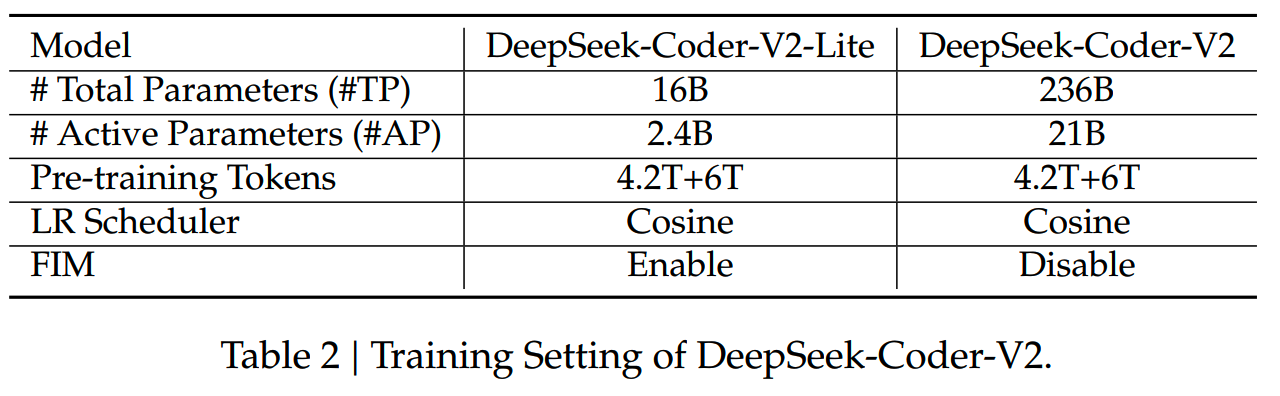
另外,为了保持模型的长文本能力,进行了长窗口的训练。用32k长度的数据,以batch size = 1152训练了1000个step,然后把长度扩展到128k,用288的batch size再训练1000个step。之后再通过YARN进行长度扩展:s=40,alpha=1,beta=32。
而在alignment阶段,使用了20k条代码相关,30k条数学相关的instruction数据,同时从DeepSeek-V2中也采样了一些通用instruction数据,构成300M token的训练数据集。
除了instruction tuning,DeepSeek-Coder-V2还进行RL。虽然代码数据可以通过执行反馈作为feedback,不过仍然可能出现部分测试case覆盖不全的情况,因此还是训练了一个reward model对生成的结果进行打分。
Qwen2.5-Coder
Qwen2.5-Coder有两个规模,1.5B和7B。
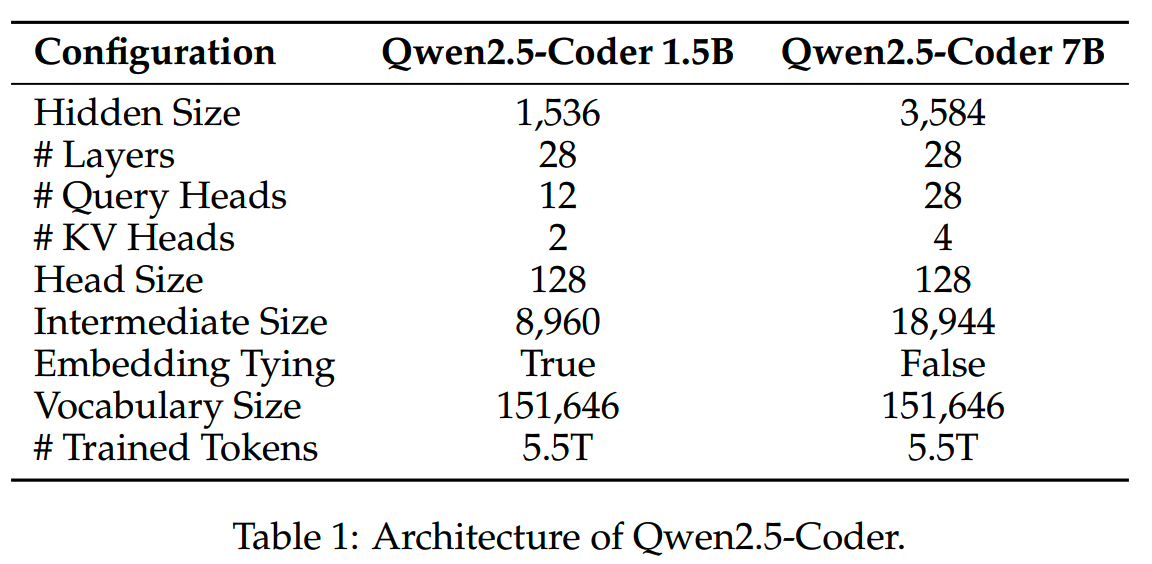
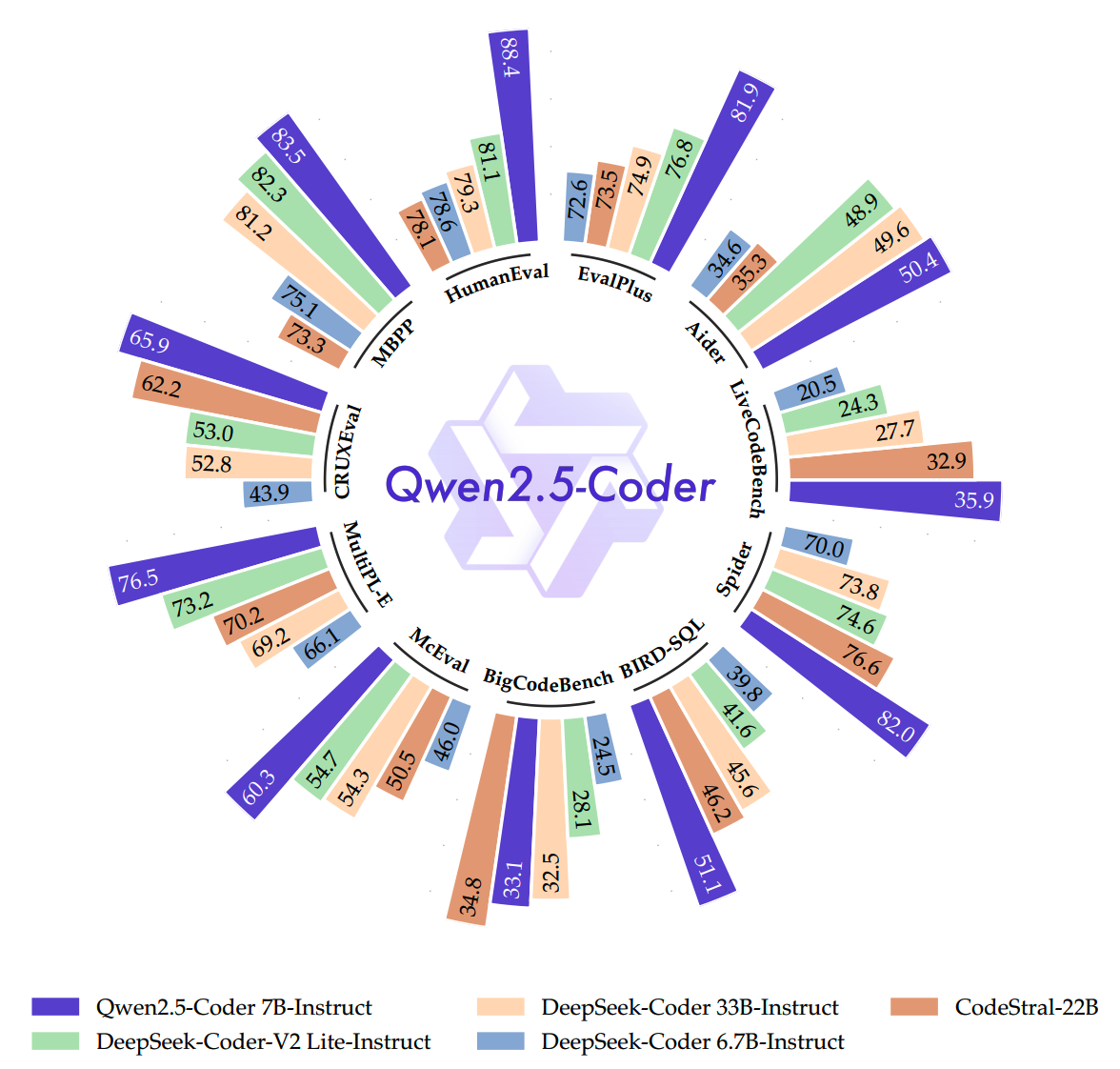
数据
Qwen2.5-Coder在Qwen2.5的基础上,又进行了5.5T数据的训练。
这些数据主要有5种:
- Source Code Data
- Text-Code Grounding Data
- Synthetic Data
- Math Data
- Text Data
1、Source Code Data
来自github 2024年2月前的数据,包括92种语言。和StarCoder2类似,也会进行一系列的rule-based处理。处理源码之外,还有Pull Requests, Commits, Jupyter Notebooks, 和 Kaggle datasets等。
2、Text-Code Grounding Data
包括代码相关的documentation, tutorials, blogs等。
这些数据使用小规模的模型(如fasttext)进行了清洗和过滤。发现更大的模型并不能带来更好的清洗效果,可能是因为小模型更加能够关注在surface-level的feature。
3、Synthetic Data
基于CodeQwen1.5生成了大量的合成数据,并通过执行反馈筛选。
4、Math Data
使用了Qwen2.5-Math的数据。
5、Text Data
从Qwen2.5的数据中抽取。会把代码相关的数据从这里面删除掉。
数据混合比例上,文中做了3中配比的实验:
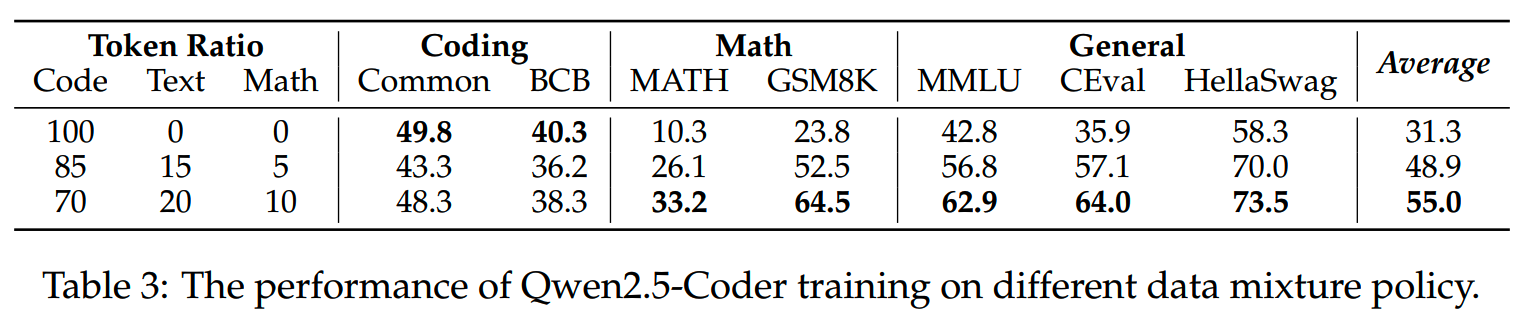
Code:Text:Math = 7:2:1的效果是最好的,比其他代码比例更高的配比效果更好。
预训练
Qwen2.5-Coder的训练流程如下:

1、File-Level Pretraining
这一阶段的训练长度为8192,使用了left-to-right和FIM两个训练目标。
tokenizer中特意增加了一些token用于标识FIM的中各个部分的位置。
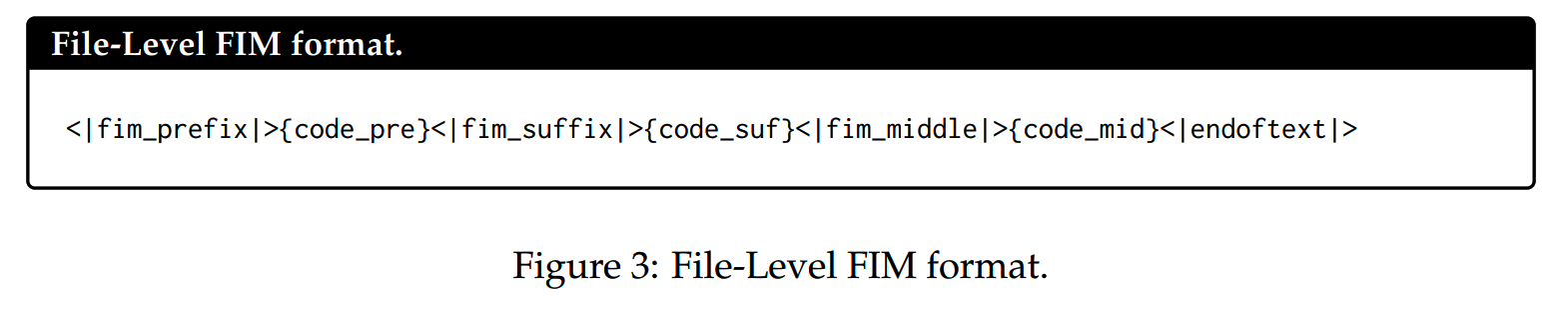
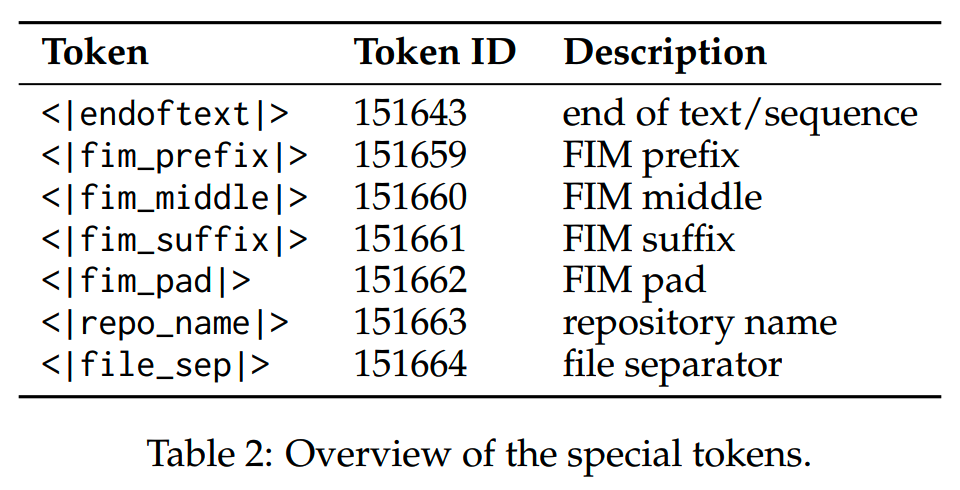
2、Repo-Level Pretraining
第二阶段是针对repo-level数据的训练。repo-level数据的一个特点就是文件多,样本长。因此需要模型具备长窗口的能力。这一阶段把RoPE的base frequency从10,000提升到1,000,000,进行了32k长度的训练,在推理时再通过YARN把窗口扩展到132k。
这个阶段训练了大约300B token的数据。FIM也从file-level改为repo-level的格式:
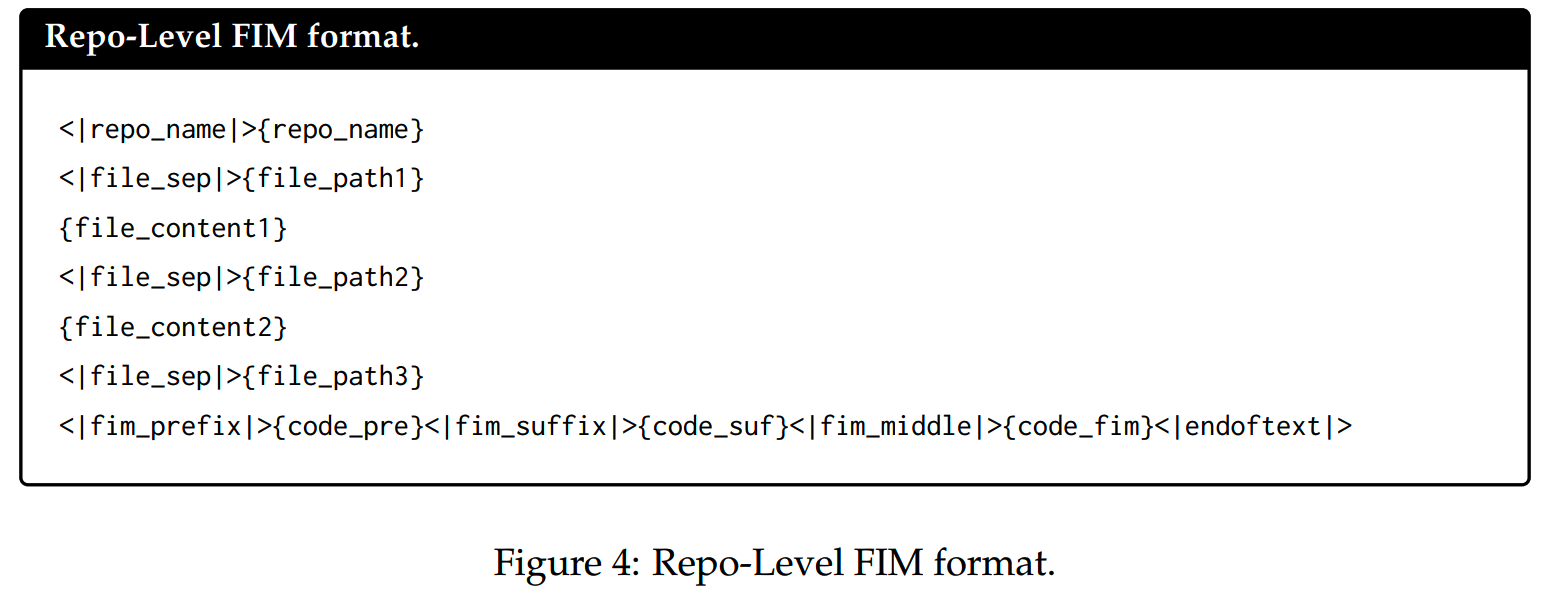
post-training
在post-training阶段,有以下关键action。
1、Multilingual Programming Code Identification
微调 CodeBERT 进行语言识别,保留主流编程语言的指令数据,随机丢弃部分长尾语言部分指令数据。对于被识别为“无编程语言”的部分数据,则大部分都会被去掉,以免影响模型的代码生成能力。
2、代码指令合成
针对 GitHub 等网上大量存在的无监督数据(代码片段),构建监督指令数据集。
具体做法是使用 LLM 根据代码片段生成指令(长度在 1024 个标记内),然后再用代码 LLM 生成响应,最后使用 LLM 评分器过滤低质量的指令和响应对,以获得最终的配对。通过这种方式,可以从不同编程语言的代码片段中构建指令数据集。
为了增加指令数据集的多样性,也可以先生成答案,然后用 LLM 评分器过滤获得最终的三元组,从而构建出具有通用代码的指令数据集。此外,还将开源指令数据集纳入到种子指令数据集中。
3、Multilingual Code Instruction Data
提出了一个multilingual multi-agent协作框架,来合成多语言指令语料库。
具体过程如下: -
创建一组特定于语言的agent,每个agent专门负责一种特定的编程语言,这些agent使用从有限的多语言指令语料库中提取的特定语言指令数据进行初始化;
-
多个语言特定的agent通过结构化对话来制定新的指令和解决方案,这个过程可以增强现有语言的能力或为新编程语言生成指令;
-
每个agent维护一个动态存储库来存储其生成历史,以避免生成相似的样本;
-
开发了一种知识蒸馏方法,使各个agent能够跨越语言边界共享内容,促进对编程概念的更全面理解;
小结
纵观几个最新的代码模型,有几点关键发现:
- FIM训练对于代码补全能力至关重要
- 代码能力和数学能力几乎总是成对出现的,这二者有很强的关联性
-
代码数据量的需求很大,需要收集尽量多的数据,而且不止需要源码,和代码相关的文档、issue、教程等都很重要
在关于代码具体怎么清洗、组织和生成上,基本上依然是各家秘而不宣的核心,而这也是我们要探索的关键点。
博客:http://www.linsight.cn/
知乎:Linsight
微信公众号:Linsight
博主微信号(添加请注明来意):
【推荐文章】
- MoE:
MoE模型的前世今生
DeepSeek-V2和MLA
昆仑万维-SkyworkMoE
成本10w刀的JetMoE
MoE的top-p
routing
对MoE模型的一些观察
从dense到MoE -- sparse
upcycling
MoE路由--expert choice
routing
- 端侧模型:
苹果智能系统模型--AFM
MiniCPM
适合移动设备的语言模型--MobileLLM
phi系列模型
Gemma2
苹果的OpenELM
bilibili的index-1.9B
- 预训练:
LLM高效预训练(一)
LLM高效预训练(二)
Llama3.1--预训练要点一览
Qwen2技术报告
Yi技术报告-划重点看细节
InternLM系列模型
GLM4报告的一些技术点
从Yuan2.0到Yuan2.0-M32
从loss视角理解大模型涌现能力
- 数据:
LLM预训练数据策略(一)
预训练数据处理--长度分解
- 长上下文:
LLM长上下文的问题
解锁大模型长上下文能力
大模型推理窗口-从有限到无限大
- 推理加速:
大模型推理加速-投机解码
大模型推理加速-MEDUSA
- 对齐:
Llama3.1--post-training要点一览
模型平均 -- model
soup
大模型偏好对齐-DPO
大模型偏好对齐-ODPO
大模型偏好对齐-simPO
大模型偏好对齐-IPO
- Transformer:
理解Attention:从起源到MHA,MQA和GQA
LLM的重复生成和ICL
transformer中normalization的二三事
从代码实现看normalization-到底做了什么
稀疏注意力计算:sliding
window attention
理解LLM位置编码:RoPE
RoPE的远距离衰减
- 项目应用:
一个模型支持智能助手系统
- CV:
CV入门--关于Vision
Transformer
CV入门--无监督学习
- 多模态:
多模态入门--CLIP
- 大模型算法题:
(1)、 (2)、 (3)、 (4)、 (5)、 (6)、 (7)、 (8)、 (9)
Reference
【1】Evaluating Large Language Models Trained on Code
https://arxiv.org/abs/2107.03374
【2】Program Synthesis with Large Language Models
https://arxiv.org/abs/2108.07732
【3】Efficient Training of Language Models to Fill in the Middle
https://arxiv.org/abs/2207.14255
【4】Code Llama: Open Foundation Models for Code
https://arxiv.org/abs/2308.12950
【5】StarCoder 2 and The Stack v2: The Next Generation
https://arxiv.org/abs/2402.19173
【6】DeepSeek-Coder-V2: Breaking the Barrier of Closed-Source Models in
Code Intelligence https://arxiv.org/abs/2406.11931
【7】Qwen2.5-Coder Technical Report https://arxiv.org/abs/2409.12186